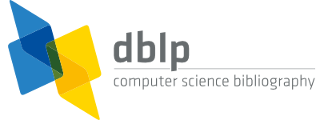


default search action
Mark van der Wilk
Person information
- affiliation: Imperial College London, UK
Refine list

refinements active!
zoomed in on ?? of ?? records
view refined list in
export refined list as
2020 – today
- 2025
- [i58]Georgia Channing, Aqib Mahfuz, Mark van der Wilk, Philip Torr, Fabio Pizzati, Christian Schröder de Witt:
PSyDUCK: Training-Free Steganography for Latent Diffusion. CoRR abs/2501.19172 (2025) - 2024
- [j5]Alexander Terenin, David R. Burt, Artem Artemev, Seth R. Flaxman, Mark van der Wilk, Carl Edward Rasmussen, Hong Ge:
Numerically Stable Sparse Gaussian Processes via Minimum Separation using Cover Trees. J. Mach. Learn. Res. 25: 26:1-26:36 (2024) - [c34]Anish Dhir, Samuel Power, Mark van der Wilk:
Bivariate Causal Discovery using Bayesian Model Selection. ICML 2024 - [c33]Seth Nabarro, Mark van der Wilk, Andrew J. Davison:
Learning in Deep Factor Graphs with Gaussian Belief Propagation. ICML 2024 - [c32]Jose Pablo Folch, Calvin Tsay, Robert M. Lee, Behrang Shafei, Weronika Ormaniec, Andreas Krause, Mark van der Wilk, Ruth Misener, Mojmir Mutny:
Transition Constrained Bayesian Optimization via Markov Decision Processes. NeurIPS 2024 - [c31]Tycho F. A. van der Ouderaa, Mark van der Wilk, Pim de Haan:
Noether's Razor: Learning Conserved Quantities. NeurIPS 2024 - [i57]Jose Pablo Folch, Calvin Tsay, Robert M. Lee, Behrang Shafei, Weronika Ormaniec, Andreas Krause, Mark van der Wilk, Ruth Misener, Mojmír Mutný:
Transition Constrained Bayesian Optimization via Markov Decision Processes. CoRR abs/2402.08406 (2024) - [i56]Sebastian W. Ober, Artem Artemev, Marcel Wagenländer, Rudolfs Grobins, Mark van der Wilk:
Recommendations for Baselines and Benchmarking Approximate Gaussian Processes. CoRR abs/2402.09849 (2024) - [i55]Ruby Sedgwick, John P. Goertz, Molly M. Stevens, Ruth Misener
, Mark van der Wilk:
Transfer Learning Bayesian Optimization to Design Competitor DNA Molecules for Use in Diagnostic Assays. CoRR abs/2402.17704 (2024) - [i54]Jixiang Qing, Becky D. Langdon, Robert M. Lee, Behrang Shafei, Mark van der Wilk, Calvin Tsay, Ruth Misener:
System-Aware Neural ODE Processes for Few-Shot Bayesian Optimization. CoRR abs/2406.02352 (2024) - [i53]Yoav Gelberg, Tycho F. A. van der Ouderaa, Mark van der Wilk, Yarin Gal:
Variational Inference Failures Under Model Symmetries: Permutation Invariant Posteriors for Bayesian Neural Networks. CoRR abs/2408.05496 (2024) - [i52]Guiomar Pescador-Barrios, Sarah Filippi, Mark van der Wilk:
"How Big is Big Enough?" Adjusting Model Size in Continual Gaussian Processes. CoRR abs/2408.07588 (2024) - [i51]Tycho F. A. van der Ouderaa, Mark van der Wilk, Pim de Haan:
Noether's razor: Learning Conserved Quantities. CoRR abs/2410.08087 (2024) - [i50]Anish Dhir, Ruby Sedgwick, Avinash Kori, Ben Glocker, Mark van der Wilk:
Continuous Bayesian Model Selection for Multivariate Causal Discovery. CoRR abs/2411.10154 (2024) - [i49]Anish Dhir, Matthew Ashman, James Requeima, Mark van der Wilk:
A Meta-Learning Approach to Bayesian Causal Discovery. CoRR abs/2412.16577 (2024) - [i48]Freddie Bickford Smith, Jannik Kossen, Eleanor Trollope, Mark van der Wilk, Adam Foster, Tom Rainforth:
Rethinking Aleatoric and Epistemic Uncertainty. CoRR abs/2412.20892 (2024) - [i47]Vincent Fortuin, Mohammad Emtiyaz Khan, Mark van der Wilk, Zoubin Ghahramani, Katharine Fisher:
Rethinking the Role of Bayesianism in the Age of Modern AI (Dagstuhl Seminar 24461). Dagstuhl Reports 14(11): 40-59 (2024) - 2023
- [j4]Jose Pablo Folch
, Robert M. Lee, Behrang Shafei, David Walz
, Calvin Tsay
, Mark van der Wilk, Ruth Misener
:
Combining multi-fidelity modelling and asynchronous batch Bayesian Optimization. Comput. Chem. Eng. 172: 108194 (2023) - [c30]Harry Jake Cunningham, Daniel Augusto de Souza, So Takao, Mark van der Wilk, Marc Peter Deisenroth:
Actually Sparse Variational Gaussian Processes. AISTATS 2023: 10395-10408 - [c29]Alexander Immer, Tycho F. A. van der Ouderaa, Mark van der Wilk, Gunnar Rätsch, Bernhard Schölkopf:
Stochastic Marginal Likelihood Gradients using Neural Tangent Kernels. ICML 2023: 14333-14352 - [c28]Tycho F. A. van der Ouderaa, Alexander Immer, Mark van der Wilk:
Learning Layer-wise Equivariances Automatically using Gradients. NeurIPS 2023 - [i46]Harry Jake Cunningham, Daniel Augusto de Souza, So Takao, Mark van der Wilk, Marc Peter Deisenroth:
Actually Sparse Variational Gaussian Processes. CoRR abs/2304.05091 (2023) - [i45]Anish Dhir, Mark van der Wilk:
Causal Discovery using Bayesian Model Selection. CoRR abs/2306.02931 (2023) - [i44]Alexander Immer, Tycho F. A. van der Ouderaa, Mark van der Wilk, Gunnar Rätsch, Bernhard Schölkopf:
Stochastic Marginal Likelihood Gradients using Neural Tangent Kernels. CoRR abs/2306.03968 (2023) - [i43]Jacob Green, Cecilia Cabrera Diaz, Maximilian A. H. Jakobs, Andrea Dimitracopoulos, Mark van der Wilk, Ryan D. Greenhalgh:
Current Methods for Drug Property Prediction in the Real World. CoRR abs/2309.17161 (2023) - [i42]Tycho F. A. van der Ouderaa, Alexander Immer, Mark van der Wilk:
Learning Layer-wise Equivariances Automatically using Gradients. CoRR abs/2310.06131 (2023) - [i41]Seth Nabarro, Mark van der Wilk, Andrew J. Davison:
Learning in Deep Factor Graphs with Gaussian Belief Propagation. CoRR abs/2311.14649 (2023) - [i40]Jose Pablo Folch, James Odgers, Shiqiang Zhang, Robert M. Lee, Behrang Shafei, David Walz, Calvin Tsay, Mark van der Wilk, Ruth Misener:
Practical Path-based Bayesian Optimization. CoRR abs/2312.00622 (2023) - [i39]Shahin Honarvar, Mark van der Wilk, Alastair F. Donaldson:
Turbulence: Systematically and Automatically Testing Instruction-Tuned Large Language Models for Code. CoRR abs/2312.14856 (2023) - 2022
- [c27]Pola Schwöbel, Martin Jørgensen, Sebastian W. Ober, Mark van der Wilk:
Last Layer Marginal Likelihood for Invariance Learning. AISTATS 2022: 3542-3555 - [c26]Vincent Fortuin
, Adrià Garriga-Alonso, Sebastian W. Ober, Florian Wenzel, Gunnar Rätsch, Richard E. Turner, Mark van der Wilk, Laurence Aitchison:
Bayesian Neural Network Priors Revisited. ICLR 2022 - [c25]Tycho F. A. van der Ouderaa, Mark van der Wilk:
Sparse Convolutions on Lie Groups. NeurReps 2022: 48-62 - [c24]Artem Artemev, Yuze An, Tilman Roeder, Mark van der Wilk:
Memory safe computations with XLA compiler. NeurIPS 2022 - [c23]Jose Pablo Folch, Shiqiang Zhang, Robert M. Lee, Behrang Shafei, David Walz, Calvin Tsay, Mark van der Wilk, Ruth Misener:
SnAKe: Bayesian Optimization with Pathwise Exploration. NeurIPS 2022 - [c22]Alexander Immer, Tycho F. A. van der Ouderaa, Gunnar Rätsch, Vincent Fortuin, Mark van der Wilk:
Invariance Learning in Deep Neural Networks with Differentiable Laplace Approximations. NeurIPS 2022 - [c21]Tycho F. A. van der Ouderaa, David W. Romero, Mark van der Wilk:
Relaxing Equivariance Constraints with Non-stationary Continuous Filters. NeurIPS 2022 - [c20]Seth Nabarro, Stoil Ganev, Adrià Garriga-Alonso, Vincent Fortuin
, Mark van der Wilk, Laurence Aitchison:
Data augmentation in Bayesian neural networks and the cold posterior effect. UAI 2022: 1434-1444 - [c19]Tycho F. A. van der Ouderaa, Mark van der Wilk:
Learning invariant weights in neural networks. UAI 2022: 1992-2001 - [i38]Jose Pablo Folch, Shiqiang Zhang, Robert M. Lee, Behrang Shafei, David Walz, Calvin Tsay, Mark van der Wilk, Ruth Misener:
SnAKe: Bayesian Optimization with Pathwise Exploration. CoRR abs/2202.00060 (2022) - [i37]Alexander Immer, Tycho F. A. van der Ouderaa, Vincent Fortuin, Gunnar Rätsch, Mark van der Wilk:
Invariance Learning in Deep Neural Networks with Differentiable Laplace Approximations. CoRR abs/2202.10638 (2022) - [i36]Tycho F. A. van der Ouderaa, Mark van der Wilk:
Learning Invariant Weights in Neural Networks. CoRR abs/2202.12439 (2022) - [i35]Tycho F. A. van der Ouderaa, David W. Romero, Mark van der Wilk:
Relaxing Equivariance Constraints with Non-stationary Continuous Filters. CoRR abs/2204.07178 (2022) - [i34]Artem Artemev, Tilman Roeder, Mark van der Wilk:
Memory Safe Computations with XLA Compiler. CoRR abs/2206.14148 (2022) - [i33]Alexander Terenin, David R. Burt, Artem Artemev, Seth R. Flaxman, Mark van der Wilk, Carl Edward Rasmussen, Hong Ge
:
Numerically Stable Sparse Gaussian Processes via Minimum Separation using Cover Trees. CoRR abs/2210.07893 (2022) - [i32]Jose Pablo Folch, Robert M. Lee, Behrang Shafei, David Walz, Calvin Tsay, Mark van der Wilk, Ruth Misener
:
Combining Multi-Fidelity Modelling and Asynchronous Batch Bayesian Optimization. CoRR abs/2211.06149 (2022) - 2021
- [j3]Vincent Fortuin, Adrià Garriga-Alonso, Mark van der Wilk, Laurence Aitchison
:
BNNpriors: A library for Bayesian neural network inference with different prior distributions. Softw. Impacts 9: 100079 (2021) - [c18]Artem Artemev, David R. Burt, Mark van der Wilk:
Tighter Bounds on the Log Marginal Likelihood of Gaussian Process Regression Using Conjugate Gradients. ICML 2021: 362-372 - [c17]Robin Ru, Clare Lyle, Lisa Schut, Miroslav Fil, Mark van der Wilk, Yarin Gal:
Speedy Performance Estimation for Neural Architecture Search. NeurIPS 2021: 4079-4092 - [c16]Vincent Dutordoir, James Hensman, Mark van der Wilk, Carl Henrik Ek, Zoubin Ghahramani, Nicolas Durrande:
Deep Neural Networks as Point Estimates for Deep Gaussian Processes. NeurIPS 2021: 9443-9455 - [c15]Sebastian W. Ober, Carl E. Rasmussen, Mark van der Wilk:
The promises and pitfalls of deep kernel learning. UAI 2021: 1206-1216 - [c14]Adrià Garriga-Alonso, Mark van der Wilk:
Correlated weights in infinite limits of deep convolutional neural networks. UAI 2021: 1998-2007 - [i31]Adrià Garriga-Alonso, Mark van der Wilk:
Correlated Weights in Infinite Limits of Deep Convolutional Neural Networks. CoRR abs/2101.04097 (2021) - [i30]Vincent Fortuin, Adrià Garriga-Alonso, Florian Wenzel, Gunnar Rätsch, Richard E. Turner, Mark van der Wilk, Laurence Aitchison:
Bayesian Neural Network Priors Revisited. CoRR abs/2102.06571 (2021) - [i29]Artem Artemev, David R. Burt, Mark van der Wilk:
Tighter Bounds on the Log Marginal Likelihood of Gaussian Process Regression Using Conjugate Gradients. CoRR abs/2102.08314 (2021) - [i28]Sebastian W. Ober, Carl E. Rasmussen, Mark van der Wilk:
The Promises and Pitfalls of Deep Kernel Learning. CoRR abs/2102.12108 (2021) - [i27]Vincent Dutordoir, Hugh Salimbeni, Eric Hambro, John McLeod, Felix Leibfried, Artem Artemev, Mark van der Wilk, James Hensman, Marc Peter Deisenroth, S. T. John:
GPflux: A Library for Deep Gaussian Processes. CoRR abs/2104.05674 (2021) - [i26]Vincent Dutordoir, James Hensman, Mark van der Wilk, Carl Henrik Ek, Zoubin Ghahramani, Nicolas Durrande:
Deep Neural Networks as Point Estimates for Deep Gaussian Processes. CoRR abs/2105.04504 (2021) - [i25]Vincent Fortuin, Adrià Garriga-Alonso, Mark van der Wilk, Laurence Aitchison:
BNNpriors: A library for Bayesian neural network inference with different prior distributions. CoRR abs/2105.06964 (2021) - [i24]Seth Nabarro, Stoil Ganev, Adrià Garriga-Alonso, Vincent Fortuin, Mark van der Wilk, Laurence Aitchison:
Data augmentation in Bayesian neural networks and the cold posterior effect. CoRR abs/2106.05586 (2021) - [i23]Pola Elisabeth Schwöbel, Martin Jørgensen, Sebastian W. Ober, Mark van der Wilk:
Last Layer Marginal Likelihood for Invariance Learning. CoRR abs/2106.07512 (2021) - [i22]Nikolaos Mourdoukoutas, Marco Federici, Georges Pantalos, Mark van der Wilk, Vincent Fortuin:
A Bayesian Approach to Invariant Deep Neural Networks. CoRR abs/2107.09301 (2021) - [i21]David R. Burt, Artem Artemev, Mark van der Wilk:
Barely Biased Learning for Gaussian Process Regression. CoRR abs/2109.09417 (2021) - 2020
- [j2]David R. Burt, Carl Edward Rasmussen, Mark van der Wilk:
Convergence of Sparse Variational Inference in Gaussian Processes Regression. J. Mach. Learn. Res. 21: 131:1-131:63 (2020) - [c13]Vincent Dutordoir, Mark van der Wilk, Artem Artemev, James Hensman:
Bayesian Image Classification with Deep Convolutional Gaussian Processes. AISTATS 2020: 1529-1539 - [c12]Clare Lyle, Lisa Schut, Robin Ru, Yarin Gal, Mark van der Wilk:
A Bayesian Perspective on Training Speed and Model Selection. NeurIPS 2020 - [c11]Miguel Monteiro, Loïc Le Folgoc, Daniel Coelho de Castro, Nick Pawlowski, Bernardo Marques, Konstantinos Kamnitsas, Mark van der Wilk, Ben Glocker:
Stochastic Segmentation Networks: Modelling Spatially Correlated Aleatoric Uncertainty. NeurIPS 2020 - [i20]Mark van der Wilk, Vincent Dutordoir, S. T. John, Artem Artemev, Vincent Adam, James Hensman:
A Framework for Interdomain and Multioutput Gaussian Processes. CoRR abs/2003.01115 (2020) - [i19]Lewis Smith, Lisa Schut, Yarin Gal, Mark van der Wilk:
Capsule Networks - A Probabilistic Perspective. CoRR abs/2004.03553 (2020) - [i18]Clare Lyle, Mark van der Wilk, Marta Kwiatkowska, Yarin Gal, Benjamin Bloem-Reddy:
On the Benefits of Invariance in Neural Networks. CoRR abs/2005.00178 (2020) - [i17]Binxin Ru, Clare Lyle, Lisa Schut, Mark van der Wilk, Yarin Gal:
Revisiting the Train Loss: an Efficient Performance Estimator for Neural Architecture Search. CoRR abs/2006.04492 (2020) - [i16]Miguel Monteiro, Loïc Le Folgoc, Daniel Coelho de Castro, Nick Pawlowski, Bernardo Marques, Konstantinos Kamnitsas, Mark van der Wilk, Ben Glocker:
Stochastic Segmentation Networks: Modelling Spatially Correlated Aleatoric Uncertainty. CoRR abs/2006.06015 (2020) - [i15]David R. Burt, Carl Edward Rasmussen, Mark van der Wilk:
Variational Orthogonal Features. CoRR abs/2006.13170 (2020) - [i14]David R. Burt, Carl Edward Rasmussen, Mark van der Wilk:
Convergence of Sparse Variational Inference in Gaussian Processes Regression. CoRR abs/2008.00323 (2020) - [i13]Clare Lyle, Lisa Schut, Binxin Ru, Yarin Gal, Mark van der Wilk:
A Bayesian Perspective on Training Speed and Model Selection. CoRR abs/2010.14499 (2020) - [i12]David R. Burt, Sebastian W. Ober, Adrià Garriga-Alonso, Mark van der Wilk:
Understanding Variational Inference in Function-Space. CoRR abs/2011.09421 (2020) - [i11]Ruby Sedgwick, John P. Goertz, Molly M. Stevens, Ruth Misener, Mark van der Wilk:
Design of Experiments for Verifying Biomolecular Networks. CoRR abs/2011.10575 (2020)
2010 – 2019
- 2019
- [b1]Mark van der Wilk:
Sparse Gaussian process approximations and applications. University of Cambridge, UK, 2019 - [c10]Mark van der Wilk, S. T. John, Artem Artemev, James Hensman:
Variational Gaussian Process Models without Matrix Inverses. AABI 2019: 1-9 - [c9]David R. Burt, Carl Edward Rasmussen, Mark van der Wilk:
Rates of Convergence for Sparse Variational Gaussian Process Regression. ICML 2019: 862-871 - [c8]Alessandro Davide Ialongo, Mark van der Wilk, James Hensman, Carl Edward Rasmussen:
Overcoming Mean-Field Approximations in Recurrent Gaussian Process Models. ICML 2019: 2931-2940 - [c7]Creighton Heaukulani, Mark van der Wilk:
Scalable Bayesian dynamic covariance modeling with variational Wishart and inverse Wishart processes. NeurIPS 2019: 4584-4594 - [c6]Dustin Tran, Mike Dusenberry, Mark van der Wilk, Danijar Hafner:
Bayesian Layers: A Module for Neural Network Uncertainty. NeurIPS 2019: 14633-14645 - [i10]Vincent Dutordoir, Mark van der Wilk, Artem Artemev, Marcin Tomczak, James Hensman:
Translation Insensitivity for Deep Convolutional Gaussian Processes. CoRR abs/1902.05888 (2019) - [i9]David R. Burt, Carl E. Rasmussen, Mark van der Wilk:
Rates of Convergence for Sparse Variational Gaussian Process Regression. CoRR abs/1903.03571 (2019) - [i8]Alessandro Davide Ialongo, Mark van der Wilk, James Hensman, Carl Edward Rasmussen:
Overcoming Mean-Field Approximations in Recurrent Gaussian Process Models. CoRR abs/1906.05828 (2019) - [i7]Creighton Heaukulani, Mark van der Wilk:
Scalable Bayesian dynamic covariance modeling with variational Wishart and inverse Wishart processes. CoRR abs/1906.09360 (2019) - 2018
- [c5]Mark van der Wilk, Matthias Bauer, S. T. John, James Hensman:
Learning Invariances using the Marginal Likelihood. NeurIPS 2018: 9960-9970 - [i6]Mark van der Wilk, Matthias Bauer, S. T. John, James Hensman:
Learning Invariances using the Marginal Likelihood. CoRR abs/1808.05563 (2018) - [i5]Alessandro Davide Ialongo, Mark van der Wilk, Carl Edward Rasmussen:
Closed-form Inference and Prediction in Gaussian Process State-Space Models. CoRR abs/1812.03580 (2018) - [i4]Dustin Tran, Michael W. Dusenberry, Mark van der Wilk, Danijar Hafner:
Bayesian Layers: A Module for Neural Network Uncertainty. CoRR abs/1812.03973 (2018) - [i3]Alessandro Davide Ialongo, Mark van der Wilk, James Hensman, Carl Edward Rasmussen:
Non-Factorised Variational Inference in Dynamical Systems. CoRR abs/1812.06067 (2018) - 2017
- [j1]Alexander G. de G. Matthews, Mark van der Wilk, Tom Nickson, Keisuke Fujii, Alexis Boukouvalas, Pablo León-Villagrá, Zoubin Ghahramani, James Hensman:
GPflow: A Gaussian Process Library using TensorFlow. J. Mach. Learn. Res. 18: 40:1-40:6 (2017) - [c4]Rowan McAllister, Yarin Gal, Alex Kendall, Mark van der Wilk, Amar Shah, Roberto Cipolla, Adrian Weller:
Concrete Problems for Autonomous Vehicle Safety: Advantages of Bayesian Deep Learning. IJCAI 2017: 4745-4753 - [c3]Mark van der Wilk, Carl Edward Rasmussen, James Hensman:
Convolutional Gaussian Processes. NIPS 2017: 2849-2858 - [i2]Mark van der Wilk, Carl Edward Rasmussen, James Hensman:
Convolutional Gaussian Processes. CoRR abs/1709.01894 (2017) - 2016
- [c2]Matthias Bauer, Mark van der Wilk, Carl Edward Rasmussen:
Understanding Probabilistic Sparse Gaussian Process Approximations. NIPS 2016: 1525-1533 - 2014
- [c1]Yarin Gal, Mark van der Wilk, Carl E. Rasmussen:
Distributed Variational Inference in Sparse Gaussian Process Regression and Latent Variable Models. NIPS 2014: 3257-3265 - [i1]Yarin Gal, Mark van der Wilk, Carl E. Rasmussen:
Distributed Variational Inference in Sparse Gaussian Process Regression and Latent Variable Models. CoRR abs/1402.1389 (2014)
Coauthor Index
aka: Carl Edward Rasmussen

manage site settings
To protect your privacy, all features that rely on external API calls from your browser are turned off by default. You need to opt-in for them to become active. All settings here will be stored as cookies with your web browser. For more information see our F.A.Q.
Unpaywalled article links
Add open access links from to the list of external document links (if available).
Privacy notice: By enabling the option above, your browser will contact the API of unpaywall.org to load hyperlinks to open access articles. Although we do not have any reason to believe that your call will be tracked, we do not have any control over how the remote server uses your data. So please proceed with care and consider checking the Unpaywall privacy policy.
Archived links via Wayback Machine
For web page which are no longer available, try to retrieve content from the of the Internet Archive (if available).
Privacy notice: By enabling the option above, your browser will contact the API of archive.org to check for archived content of web pages that are no longer available. Although we do not have any reason to believe that your call will be tracked, we do not have any control over how the remote server uses your data. So please proceed with care and consider checking the Internet Archive privacy policy.
Reference lists
Add a list of references from ,
, and
to record detail pages.
load references from crossref.org and opencitations.net
Privacy notice: By enabling the option above, your browser will contact the APIs of crossref.org, opencitations.net, and semanticscholar.org to load article reference information. Although we do not have any reason to believe that your call will be tracked, we do not have any control over how the remote server uses your data. So please proceed with care and consider checking the Crossref privacy policy and the OpenCitations privacy policy, as well as the AI2 Privacy Policy covering Semantic Scholar.
Citation data
Add a list of citing articles from and
to record detail pages.
load citations from opencitations.net
Privacy notice: By enabling the option above, your browser will contact the API of opencitations.net and semanticscholar.org to load citation information. Although we do not have any reason to believe that your call will be tracked, we do not have any control over how the remote server uses your data. So please proceed with care and consider checking the OpenCitations privacy policy as well as the AI2 Privacy Policy covering Semantic Scholar.
OpenAlex data
Load additional information about publications from .
Privacy notice: By enabling the option above, your browser will contact the API of openalex.org to load additional information. Although we do not have any reason to believe that your call will be tracked, we do not have any control over how the remote server uses your data. So please proceed with care and consider checking the information given by OpenAlex.
last updated on 2025-04-30 20:45 CEST by the dblp team
all metadata released as open data under CC0 1.0 license
see also: Terms of Use | Privacy Policy | Imprint