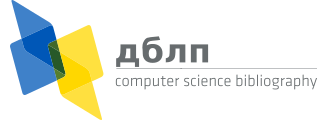


Остановите войну!
for scientists:


default search action
Preetum Nakkiran
Person information
Refine list

refinements active!
zoomed in on ?? of ?? records
view refined list in
export refined list as
2020 – today
- 2024
- [c23]Vimal Thilak, Chen Huang, Omid Saremi, Laurent Dinh, Hanlin Goh, Preetum Nakkiran, Joshua M. Susskind, Etai Littwin:
LiDAR: Sensing Linear Probing Performance in Joint Embedding SSL Architectures. ICLR 2024 - [c22]Jaroslaw Blasiok, Parikshit Gopalan, Lunjia Hu, Adam Tauman Kalai, Preetum Nakkiran:
Loss Minimization Yields Multicalibration for Large Neural Networks. ITCS 2024: 17:1-17:21 - [i37]Dutch Hansen, Siddartha Devic, Preetum Nakkiran, Vatsal Sharan:
When is Multicalibration Post-Processing Necessary? CoRR abs/2406.06487 (2024) - [i36]Preetum Nakkiran, Arwen Bradley, Hattie Zhou, Madhu Advani:
Step-by-Step Diffusion: An Elementary Tutorial. CoRR abs/2406.08929 (2024) - 2023
- [c21]Gal Kaplun, Nikhil Ghosh, Saurabh Garg, Boaz Barak, Preetum Nakkiran:
Deconstructing Distributions: A Pointwise Framework of Learning. ICLR 2023 - [c20]Jaroslaw Blasiok, Parikshit Gopalan, Lunjia Hu, Preetum Nakkiran:
When Does Optimizing a Proper Loss Yield Calibration? NeurIPS 2023 - [c19]Jaroslaw Blasiok
, Parikshit Gopalan, Lunjia Hu
, Preetum Nakkiran:
A Unifying Theory of Distance from Calibration. STOC 2023: 1727-1740 - [i35]Jaroslaw Blasiok
, Parikshit Gopalan, Lunjia Hu, Adam Tauman Kalai, Preetum Nakkiran:
Loss minimization yields multicalibration for large neural networks. CoRR abs/2304.09424 (2023) - [i34]Jaroslaw Blasiok, Parikshit Gopalan, Lunjia Hu, Preetum Nakkiran:
When Does Optimizing a Proper Loss Yield Calibration? CoRR abs/2305.18764 (2023) - [i33]Jaroslaw Blasiok
, Preetum Nakkiran:
Smooth ECE: Principled Reliability Diagrams via Kernel Smoothing. CoRR abs/2309.12236 (2023) - [i32]Hattie Zhou, Arwen Bradley, Etai Littwin, Noam Razin, Omid Saremi, Josh M. Susskind, Samy Bengio, Preetum Nakkiran:
What Algorithms can Transformers Learn? A Study in Length Generalization. CoRR abs/2310.16028 (2023) - [i31]Noam Razin, Hattie Zhou, Omid Saremi, Vimal Thilak, Arwen Bradley, Preetum Nakkiran, Joshua M. Susskind, Etai Littwin:
Vanishing Gradients in Reinforcement Finetuning of Language Models. CoRR abs/2310.20703 (2023) - [i30]Vimal Thilak, Chen Huang, Omid Saremi, Laurent Dinh, Hanlin Goh, Preetum Nakkiran, Joshua M. Susskind, Etai Littwin:
LiDAR: Sensing Linear Probing Performance in Joint Embedding SSL Architectures. CoRR abs/2312.04000 (2023) - [i29]Micah Goldblum, Anima Anandkumar, Richard G. Baraniuk, Tom Goldstein, Kyunghyun Cho, Zachary C. Lipton, Melanie Mitchell, Preetum Nakkiran, Max Welling, Andrew Gordon Wilson:
Perspectives on the State and Future of Deep Learning - 2023. CoRR abs/2312.09323 (2023) - 2022
- [j2]Jaroslaw Blasiok
, Venkatesan Guruswami
, Preetum Nakkiran, Atri Rudra, Madhu Sudan:
General Strong Polarization. J. ACM 69(2): 11:1-11:67 (2022) - [j1]Pasin Manurangsi, Preetum Nakkiran, Luca Trevisan:
Near-Optimal NP-Hardness of Approximating Max k-CSPR. Theory Comput. 18: 1-29 (2022) - [c18]Gal Kaplun, Eran Malach, Preetum Nakkiran, Shai Shalev-Shwartz:
Knowledge Distillation: Bad Models Can Be Good Role Models. NeurIPS 2022 - [c17]Bogdan Kulynych, Yao-Yuan Yang, Yaodong Yu, Jaroslaw Blasiok, Preetum Nakkiran:
What You See is What You Get: Principled Deep Learning via Distributional Generalization. NeurIPS 2022 - [c16]Neil Mallinar, James B. Simon, Amirhesam Abedsoltan, Parthe Pandit, Misha Belkin, Preetum Nakkiran:
Benign, Tempered, or Catastrophic: Toward a Refined Taxonomy of Overfitting. NeurIPS 2022 - [i28]Like Hui, Mikhail Belkin, Preetum Nakkiran:
Limitations of Neural Collapse for Understanding Generalization in Deep Learning. CoRR abs/2202.08384 (2022) - [i27]Gal Kaplun, Nikhil Ghosh, Saurabh Garg, Boaz Barak, Preetum Nakkiran:
Deconstructing Distributions: A Pointwise Framework of Learning. CoRR abs/2202.09931 (2022) - [i26]Gal Kaplun, Eran Malach, Preetum Nakkiran, Shai Shalev-Shwartz:
Knowledge Distillation: Bad Models Can Be Good Role Models. CoRR abs/2203.14649 (2022) - [i25]Bogdan Kulynych, Yao-Yuan Yang, Yaodong Yu, Jaroslaw Blasiok
, Preetum Nakkiran:
What You See is What You Get: Distributional Generalization for Algorithm Design in Deep Learning. CoRR abs/2204.03230 (2022) - [i24]Nikhil Vyas, Yamini Bansal, Preetum Nakkiran:
Limitations of the NTK for Understanding Generalization in Deep Learning. CoRR abs/2206.10012 (2022) - [i23]Neil Mallinar, James B. Simon, Amirhesam Abedsoltan, Parthe Pandit, Mikhail Belkin, Preetum Nakkiran:
Benign, Tempered, or Catastrophic: A Taxonomy of Overfitting. CoRR abs/2207.06569 (2022) - [i22]A. Michael Carrell, Neil Mallinar, James Lucas, Preetum Nakkiran:
The Calibration Generalization Gap. CoRR abs/2210.01964 (2022) - [i21]Elan Rosenfeld, Preetum Nakkiran, Hadi Pouransari, Oncel Tuzel, Fartash Faghri:
APE: Aligning Pretrained Encoders to Quickly Learn Aligned Multimodal Representations. CoRR abs/2210.03927 (2022) - [i20]Jaroslaw Blasiok
, Parikshit Gopalan, Lunjia Hu, Preetum Nakkiran:
A Unifying Theory of Distance from Calibration. CoRR abs/2211.16886 (2022) - 2021
- [c15]Preetum Nakkiran, Behnam Neyshabur, Hanie Sedghi:
The Deep Bootstrap Framework: Good Online Learners are Good Offline Generalizers. ICLR 2021 - [c14]Preetum Nakkiran, Prayaag Venkat, Sham M. Kakade, Tengyu Ma:
Optimal Regularization can Mitigate Double Descent. ICLR 2021 - [c13]Yamini Bansal, Preetum Nakkiran, Boaz Barak:
Revisiting Model Stitching to Compare Neural Representations. NeurIPS 2021: 225-236 - [i19]Yamini Bansal, Preetum Nakkiran, Boaz Barak:
Revisiting Model Stitching to Compare Neural Representations. CoRR abs/2106.07682 (2021) - [i18]Preetum Nakkiran:
Turing-Universal Learners with Optimal Scaling Laws. CoRR abs/2111.05321 (2021) - 2020
- [c12]Preetum Nakkiran, Gal Kaplun, Yamini Bansal, Tristan Yang, Boaz Barak, Ilya Sutskever:
Deep Double Descent: Where Bigger Models and More Data Hurt. ICLR 2020 - [i17]Preetum Nakkiran, Prayaag Venkat, Sham M. Kakade, Tengyu Ma:
Optimal Regularization Can Mitigate Double Descent. CoRR abs/2003.01897 (2020) - [i16]Preetum Nakkiran:
Learning Rate Annealing Can Provably Help Generalization, Even for Convex Problems. CoRR abs/2005.07360 (2020) - [i15]Preetum Nakkiran, Yamini Bansal:
Distributional Generalization: A New Kind of Generalization. CoRR abs/2009.08092 (2020) - [i14]Preetum Nakkiran, Behnam Neyshabur, Hanie Sedghi:
The Deep Bootstrap: Good Online Learners are Good Offline Generalizers. CoRR abs/2010.08127 (2020)
2010 – 2019
- 2019
- [c11]Chi-Ning Chou, Zhixian Lei, Preetum Nakkiran:
Tracking the l2 Norm with Constant Update Time. APPROX-RANDOM 2019: 2:1-2:15 - [c10]Akshay Degwekar, Preetum Nakkiran, Vinod Vaikuntanathan:
Computational Limitations in Robust Classification and Win-Win Results. COLT 2019: 994-1028 - [c9]Venkatesan Guruswami, Preetum Nakkiran, Madhu Sudan:
Algorithmic Polarization for Hidden Markov Models. ITCS 2019: 39:1-39:19 - [c8]Dimitris Kalimeris, Gal Kaplun, Preetum Nakkiran, Benjamin L. Edelman, Tristan Yang, Boaz Barak, Haofeng Zhang:
SGD on Neural Networks Learns Functions of Increasing Complexity. NeurIPS 2019: 3491-3501 - [i13]Preetum Nakkiran:
Adversarial Robustness May Be at Odds With Simplicity. CoRR abs/1901.00532 (2019) - [i12]Preetum Nakkiran, Gal Kaplun, Dimitris Kalimeris, Tristan Yang, Benjamin L. Edelman
, Fred Zhang, Boaz Barak:
SGD on Neural Networks Learns Functions of Increasing Complexity. CoRR abs/1905.11604 (2019) - [i11]Preetum Nakkiran, Gal Kaplun, Yamini Bansal, Tristan Yang, Boaz Barak, Ilya Sutskever:
Deep Double Descent: Where Bigger Models and More Data Hurt. CoRR abs/1912.02292 (2019) - [i10]Preetum Nakkiran:
More Data Can Hurt for Linear Regression: Sample-wise Double Descent. CoRR abs/1912.07242 (2019) - 2018
- [c7]Jaroslaw Blasiok
, Venkatesan Guruswami, Preetum Nakkiran, Atri Rudra, Madhu Sudan:
General strong polarization. STOC 2018: 485-492 - [i9]Jaroslaw Blasiok, Venkatesan Guruswami, Preetum Nakkiran, Atri Rudra, Madhu Sudan:
General Strong Polarization. CoRR abs/1802.02718 (2018) - [i8]Chi-Ning Chou, Zhixian Lei, Preetum Nakkiran:
Tracking the 𝓁2 Norm with Constant Update Time. CoRR abs/1807.06479 (2018) - [i7]Preetum Nakkiran, Jaroslaw Blasiok:
The Generic Holdout: Preventing False-Discoveries in Adaptive Data Science. CoRR abs/1809.05596 (2018) - [i6]Venkatesan Guruswami, Preetum Nakkiran, Madhu Sudan:
Algorithmic Polarization for Hidden Markov Models. CoRR abs/1810.01969 (2018) - [i5]Jaroslaw Blasiok, Venkatesan Guruswami, Preetum Nakkiran, Atri Rudra, Madhu Sudan:
General Strong Polarization. Electron. Colloquium Comput. Complex. TR18 (2018) - 2017
- [i4]Charalampos E. Tsourakakis, Michael Mitzenmacher, Jaroslaw Blasiok, Ben Lawson, Preetum Nakkiran, Vasileios Nakos:
Predicting Positive and Negative Links with Noisy Queries: Theory & Practice. CoRR abs/1709.07308 (2017) - 2016
- [c6]Pasin Manurangsi, Preetum Nakkiran, Luca Trevisan
:
Near-Optimal UGC-hardness of Approximating Max k-CSP_R. APPROX-RANDOM 2016: 15:1-15:28 - [c5]Preetum Nakkiran, K. V. Rashmi, Kannan Ramchandran:
Optimal systematic distributed storage codes with fast encoding. ISIT 2016: 430-434 - 2015
- [c4]K. V. Rashmi, Preetum Nakkiran, Jingyan Wang, Nihar B. Shah, Kannan Ramchandran:
Having Your Cake and Eating It Too: Jointly Optimal Erasure Codes for I/O, Storage, and Network-bandwidth. FAST 2015: 81-94 - [c3]Rohit Prabhavalkar, Raziel Alvarez, Carolina Parada, Preetum Nakkiran, Tara N. Sainath:
Automatic gain control and multi-style training for robust small-footprint keyword spotting with deep neural networks. ICASSP 2015: 4704-4708 - [c2]Preetum Nakkiran, Raziel Alvarez, Rohit Prabhavalkar
, Carolina Parada:
Compressing deep neural networks using a rank-constrained topology. INTERSPEECH 2015: 1473-1477 - [i3]Preetum Nakkiran, K. V. Rashmi, Kannan Ramchandran:
Optimal Systematic Distributed Storage Codes with Fast Encoding. CoRR abs/1509.01858 (2015) - [i2]Pasin Manurangsi, Preetum Nakkiran, Luca Trevisan:
Near-Optimal UGC-hardness of Approximating Max k-CSP_R. CoRR abs/1511.06558 (2015) - 2014
- [c1]Preetum Nakkiran, Nihar B. Shah, K. V. Rashmi:
Fundamental limits on communication for oblivious updates in storage networks. GLOBECOM 2014: 2363-2368 - [i1]Preetum Nakkiran, Nihar B. Shah, K. V. Rashmi:
Fundamental Limits on Communication for Oblivious Updates in Storage Networks. CoRR abs/1409.1666 (2014)
Coauthor Index

manage site settings
To protect your privacy, all features that rely on external API calls from your browser are turned off by default. You need to opt-in for them to become active. All settings here will be stored as cookies with your web browser. For more information see our F.A.Q.
Unpaywalled article links
Add open access links from to the list of external document links (if available).
Privacy notice: By enabling the option above, your browser will contact the API of unpaywall.org to load hyperlinks to open access articles. Although we do not have any reason to believe that your call will be tracked, we do not have any control over how the remote server uses your data. So please proceed with care and consider checking the Unpaywall privacy policy.
Archived links via Wayback Machine
For web page which are no longer available, try to retrieve content from the of the Internet Archive (if available).
Privacy notice: By enabling the option above, your browser will contact the API of archive.org to check for archived content of web pages that are no longer available. Although we do not have any reason to believe that your call will be tracked, we do not have any control over how the remote server uses your data. So please proceed with care and consider checking the Internet Archive privacy policy.
Reference lists
Add a list of references from ,
, and
to record detail pages.
load references from crossref.org and opencitations.net
Privacy notice: By enabling the option above, your browser will contact the APIs of crossref.org, opencitations.net, and semanticscholar.org to load article reference information. Although we do not have any reason to believe that your call will be tracked, we do not have any control over how the remote server uses your data. So please proceed with care and consider checking the Crossref privacy policy and the OpenCitations privacy policy, as well as the AI2 Privacy Policy covering Semantic Scholar.
Citation data
Add a list of citing articles from and
to record detail pages.
load citations from opencitations.net
Privacy notice: By enabling the option above, your browser will contact the API of opencitations.net and semanticscholar.org to load citation information. Although we do not have any reason to believe that your call will be tracked, we do not have any control over how the remote server uses your data. So please proceed with care and consider checking the OpenCitations privacy policy as well as the AI2 Privacy Policy covering Semantic Scholar.
OpenAlex data
Load additional information about publications from .
Privacy notice: By enabling the option above, your browser will contact the API of openalex.org to load additional information. Although we do not have any reason to believe that your call will be tracked, we do not have any control over how the remote server uses your data. So please proceed with care and consider checking the information given by OpenAlex.
last updated on 2024-07-29 21:30 CEST by the dblp team
all metadata released as open data under CC0 1.0 license
see also: Terms of Use | Privacy Policy | Imprint