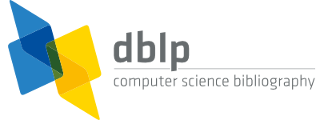


default search action
Cynthia Rudin
Person information
- affiliation: Duke University, USA
Refine list

refinements active!
zoomed in on ?? of ?? records
view refined list in
export refined list as
2020 – today
- 2025
- [c96]Yingfan Wang, Yiyang Sun, Haiyang Huang, Cynthia Rudin:
Dimension Reduction with Locally Adjusted Graphs. AAAI 2025: 21357-21365 - [c95]Ishaan Maitra, Raymond Lin, Eric Chen, Jon Donnelly, Sanja Scepanovic, Cynthia Rudin:
How Your Location Relates to Health: Variable Importance and Interpretable Machine Learning for Environmental and Sociodemographic Data. AAAI 2025: 28240-28248 - [i118]Ishaan Maitra, Raymond Lin, Eric Chen, Jon Donnelly, Sanja Scepanovic, Cynthia Rudin:
How Your Location Relates to Health: Variable Importance and Interpretable Machine Learning for Environmental and Sociodemographic Data. CoRR abs/2501.02111 (2025) - [i117]Varun Babbar, Hayden McTavish, Cynthia Rudin, Margo I. Seltzer:
Near Optimal Decision Trees in a SPLIT Second. CoRR abs/2502.15988 (2025) - [i116]Chudi Zhong, Panyu Chen, Cynthia Rudin:
Models That Are Interpretable But Not Transparent. CoRR abs/2502.19502 (2025) - [i115]Jon Donnelly, Zhicheng Guo, Alina Jade Barnett, Hayden McTavish, Chaofan Chen, Cynthia Rudin:
Rashomon Sets for Prototypical-Part Networks: Editing Interpretable Models in Real-Time. CoRR abs/2503.01087 (2025) - 2024
- [j52]Manickam Ashokkumar
, Wenwen Mei
, Jackson J. Peterson
, Yuriko Harigaya
, David M. Murdoch
, David M. Margolis
, Caleb Kornfein
, Alexander X. Oesterling
, Zhicheng Guo
, Cynthia Rudin
, Yuchao Jiang
, Edward P. Browne
:
Integrated Single-cell Multiomic Analysis of HIV Latency Reversal Reveals Novel Regulators of Viral Reactivation. Genom. Proteom. Bioinform. 22(1) (2024) - [j51]Sully F. Chen
, Zhicheng Guo, Cheng Ding, Xiao Hu, Cynthia Rudin
:
Sparse learned kernels for interpretable and efficient medical time series processing. Nat. Mac. Intell. 6(10): 1132-1144 (2024) - [j50]Cheng Ding
, Zhicheng Guo
, Cynthia Rudin
, Ran Xiao
, Amit J. Shah
, Duc H. Do
, Randall J. Lee, Gari D. Clifford
, Fadi B. Nahab
, Xiao Hu
:
Learning From Alarms: A Robust Learning Approach for Accurate Photoplethysmography-Based Atrial Fibrillation Detection Using Eight Million Samples Labeled With Imprecise Arrhythmia Alarms. IEEE J. Biomed. Health Informatics 28(5): 2650-2661 (2024) - [c94]Travis Seale-Carlisle, Saksham Jain, Courtney Lee, Caroline Levenson, Swathi Ramprasad, Brandon Garrett, Sudeepa Roy, Cynthia Rudin, Alexander Volfovsky:
Evaluating Pre-trial Programs Using Interpretable Machine Learning Matching Algorithms for Causal Inference. AAAI 2024: 22331-22340 - [c93]Rui Zhang, Rui Xin, Margo I. Seltzer, Cynthia Rudin:
Optimal Sparse Survival Trees. AISTATS 2024: 352-360 - [c92]Yiyang Sun, Zhi Chen, Vittorio Orlandi, Tong Wang, Cynthia Rudin:
Sparse and Faithful Explanations Without Sparse Models. AISTATS 2024: 2071-2079 - [c91]Harsh Parikh, Quinn Lanners, Zade Akras, Sahar Zafar, M. Brandon Westover, Cynthia Rudin, Alexander Volfovsky:
Safe and Interpretable Estimation of Optimal Treatment Regimes. AISTATS 2024: 2134-2142 - [c90]Srikar Katta, Harsh Parikh, Cynthia Rudin, Alexander Volfovsky:
Interpretable Causal Inference for Analyzing Wearable, Sensor, and Distributional Data. AISTATS 2024: 3340-3348 - [c89]Julia Yang, Alina Jade Barnett, Jon Donnelly, Satvik Kishore, Jerry Fang, Fides Regina Schwartz, Chaofan Chen, Joseph Y. Lo, Cynthia Rudin:
FPN-IAIA-BL: A Multi-Scale Interpretable Deep Learning Model for Classification of Mass Margins in Digital Mammography. CVPR Workshops 2024: 5003-5009 - [c88]Cynthia Rudin, Chudi Zhong, Lesia Semenova, Margo I. Seltzer, Ronald Parr, Jiachang Liu, Srikar Katta, Jon Donnelly, Harry Chen, Zachery Boner:
Position: Amazing Things Come From Having Many Good Models. ICML 2024 - [c87]Stephen Ni-Hahn, Weihan Xu, Zirui Yin, Rico Zhu, Simon Mak, Yue Jiang, Cynthia Rudin:
A New Dataset, Notation Software, and Representation for Computational Schenkerian Analysis. ISMIR 2024: 866-873 - [c86]Stephen Hahn
, Jerry Yin
, Rico Zhu
, Weihan Xu
, Yue Jiang
, Simon Mak
, Cynthia Rudin
:
SentHYMNent: An Interpretable and Sentiment-Driven Model for Algorithmic Melody Harmonization. KDD 2024: 5050-5060 - [c85]Jiachang Liu, Rui Zhang, Cynthia Rudin:
FastSurvival: Hidden Computational Blessings in Training Cox Proportional Hazards Models. NeurIPS 2024 - [c84]Haiyang Huang, Yingfan Wang, Cynthia Rudin:
Navigating the Effect of Parametrization for Dimensionality Reduction. NeurIPS 2024 - [c83]Zachery Boner, Harry Chen, Lesia Semenova, Ronald Parr, Cynthia Rudin:
Using Noise to Infer Aspects of Simplicity Without Learning. NeurIPS 2024 - [c82]Chiyu Ma, Jon Donnelly, Wenjun Liu, Soroush Vosoughi, Cynthia Rudin, Chaofan Chen:
Interpretable Image Classification with Adaptive Prototype-based Vision Transformers. NeurIPS 2024 - [c81]Hayden McTavish, Jon Donnelly, Margo I. Seltzer, Cynthia Rudin:
Interpretable Generalized Additive Models for Datasets with Missing Values. NeurIPS 2024 - [c80]Yiyang Sun, Tong Wang, Cynthia Rudin:
Improving Decision Sparsity. NeurIPS 2024 - [i114]Rui Zhang, Rui Xin, Margo I. Seltzer, Cynthia Rudin:
Optimal Sparse Survival Trees. CoRR abs/2401.15330 (2024) - [i113]Yiyang Sun, Zhi Chen, Vittorio Orlandi, Tong Wang, Cynthia Rudin:
Sparse and Faithful Explanations Without Sparse Models. CoRR abs/2402.09702 (2024) - [i112]Varun Babbar, Zhicheng Guo, Cynthia Rudin:
What is different between these datasets? CoRR abs/2403.05652 (2024) - [i111]Elita A. Lobo, Harvineet Singh, Marek Petrik, Cynthia Rudin, Himabindu Lakkaraju:
Data Poisoning Attacks on Off-Policy Policy Evaluation Methods. CoRR abs/2404.04714 (2024) - [i110]Cheng Ding, Zhicheng Guo, Zhaoliang Chen, Randall J. Lee, Cynthia Rudin, Xiao Hu:
SiamQuality: A ConvNet-Based Foundation Model for Imperfect Physiological Signals. CoRR abs/2404.17667 (2024) - [i109]Julia Yang
, Alina Jade Barnett
, Jon Donnelly, Satvik Kishore, Jerry Fang, Fides Regina Schwartz, Chaofan Chen, Joseph Y. Lo, Cynthia Rudin:
FPN-IAIA-BL: A Multi-Scale Interpretable Deep Learning Model for Classification of Mass Margins in Digital Mammography. CoRR abs/2406.06386 (2024) - [i108]Frank Willard, Luke Moffett, Emmanuel Mokel, Jon Donnelly, Stark Guo, Julia Yang, Giyoung Kim, Alina Jade Barnett
, Cynthia Rudin:
This Looks Better than That: Better Interpretable Models with ProtoPNeXt. CoRR abs/2406.14675 (2024) - [i107]Cynthia Rudin, Chudi Zhong, Lesia Semenova, Margo I. Seltzer, Ronald Parr, Jiachang Liu, Srikar Katta, Jon Donnelly, Harry Chen, Zachery Boner:
Amazing Things Come From Having Many Good Models. CoRR abs/2407.04846 (2024) - [i106]Stephen Ni-Hahn, Weihan Xu, Jerry Yin, Rico Zhu, Simon Mak, Yue Jiang, Cynthia Rudin:
A New Dataset, Notation Software, and Representation for Computational Schenkerian Analysis. CoRR abs/2408.07184 (2024) - [i105]Mary Bastawrous, Zhi Chen, Alexander C. Ogren, Chiara Daraio, Cynthia Rudin, L. Catherine Brinson:
Phononic materials with effectively scale-separated hierarchical features using interpretable machine learning. CoRR abs/2408.08428 (2024) - [i104]Jiachang Liu, Rui Zhang, Cynthia Rudin:
FastSurvival: Hidden Computational Blessings in Training Cox Proportional Hazards Models. CoRR abs/2410.19081 (2024) - [i103]Yiyang Sun, Tong Wang, Cynthia Rudin:
Improving Decision Sparsity. CoRR abs/2410.20483 (2024) - [i102]Chiyu Ma, Jon Donnelly, Wenjun Liu, Soroush Vosoughi, Cynthia Rudin, Chaofan Chen:
Interpretable Image Classification with Adaptive Prototype-based Vision Transformers. CoRR abs/2410.20722 (2024) - [i101]Haiyang Huang, Yingfan Wang, Cynthia Rudin:
Navigating the Effect of Parametrization for Dimensionality Reduction. CoRR abs/2411.15894 (2024) - [i100]Hayden McTavish, Jon Donnelly, Margo I. Seltzer, Cynthia Rudin:
Interpretable Generalized Additive Models for Datasets with Missing Values. CoRR abs/2412.02646 (2024) - [i99]Yingfan Wang, Yiyang Sun, Haiyang Huang, Cynthia Rudin:
Dimension Reduction with Locally Adjusted Graphs. CoRR abs/2412.15426 (2024) - 2023
- [j49]Lina Zhou, Cynthia Rudin, Matthew C. Gombolay, Jim Spohrer, Michelle Zhou, Souren Paul:
From Artificial Intelligence (AI) to Intelligence Augmentation (IA): Design Principles, Potential Risks, and Emerging Issues. AIS Trans. Hum. Comput. Interact. 15(1): 111-135 (2023) - [j48]Cynthia Rudin, Yaron Shaposhnik:
Globally-Consistent Rule-Based Summary-Explanations for Machine Learning Models: Application to Credit-Risk Evaluation. J. Mach. Learn. Res. 24: 16:1-16:44 (2023) - [c79]Rui Zhang, Rui Xin, Margo I. Seltzer, Cynthia Rudin:
Optimal Sparse Regression Trees. AAAI 2023: 11270-11279 - [c78]Edwin Agnew, Michelle Qiu, Lily Zhu, Sam Wiseman, Cynthia Rudin:
The Mechanical Bard: An Interpretable Machine Learning Approach to Shakespearean Sonnet Generation. ACL (2) 2023: 1627-1638 - [c77]Zhi Chen, Sarah Tan, Urszula Chajewska, Cynthia Rudin, Rich Caruana:
Missing Values and Imputation in Healthcare Data: Can Interpretable Machine Learning Help? CHIL 2023: 86-99 - [c76]Stephen Hahn
, Rico Zhu
, Simon Mak
, Cynthia Rudin
, Yue Jiang
:
An Interpretable, Flexible, and Interactive Probabilistic Framework for Melody Generation. KDD 2023: 4089-4099 - [c75]Yanchen Jessie Ou, Alina Jade Barnett
, Anika Mitra, Fides Regina Schwartz
, Chaofan Chen, Lars J. Grimm, Joseph Y. Lo, Cynthia Rudin:
A user interface to communicate interpretable AI decisions to radiologists. Image Perception, Observer Performance, and Technology Assessment 2023 - [c74]Yanchen Ou, Alina Jade Barnett, Anika Mitra, Fides Regina Schwartz, Chaofan Chen, Lars J. Grimm, Joseph Y. Lo, Cynthia Rudin:
A user interface to communicate interpretable AI decisions to radiologists (Erratum). Image Perception, Observer Performance, and Technology Assessment 2023 - [c73]Jiachang Liu, Sam Rosen, Chudi Zhong, Cynthia Rudin:
OKRidge: Scalable Optimal k-Sparse Ridge Regression. NeurIPS 2023 - [c72]Jon Donnelly, Srikar Katta, Cynthia Rudin, Edward P. Browne:
The Rashomon Importance Distribution: Getting RID of Unstable, Single Model-based Variable Importance. NeurIPS 2023 - [c71]Chiyu Ma, Brandon Zhao, Chaofan Chen, Cynthia Rudin:
This Looks Like Those: Illuminating Prototypical Concepts Using Multiple Visualizations. NeurIPS 2023 - [c70]Lesia Semenova, Harry Chen, Ronald Parr, Cynthia Rudin:
A Path to Simpler Models Starts With Noise. NeurIPS 2023 - [c69]Chudi Zhong, Zhi Chen, Jiachang Liu, Margo I. Seltzer, Cynthia Rudin:
Exploring and Interacting with the Set of Good Sparse Generalized Additive Models. NeurIPS 2023 - [c68]Quinn Lanners, Harsh Parikh, Alexander Volfovsky, Cynthia Rudin, David Page:
Variable importance matching for causal inference. UAI 2023: 1174-1184 - [i98]Quinn Lanners, Harsh Parikh, Alexander Volfovsky, Cynthia Rudin, David Page:
From Feature Importance to Distance Metric: An Almost Exact Matching Approach for Causal Inference. CoRR abs/2302.11715 (2023) - [i97]Zhi Chen, Chudi Zhong, Margo I. Seltzer, Cynthia Rudin:
Understanding and Exploring the Whole Set of Good Sparse Generalized Additive Models. CoRR abs/2303.16047 (2023) - [i96]Jiachang Liu, Sam Rosen, Chudi Zhong, Cynthia Rudin:
OKRidge: Scalable Optimal k-Sparse Ridge Regression for Learning Dynamical Systems. CoRR abs/2304.06686 (2023) - [i95]Zhi Chen, Sarah Tan, Urszula Chajewska, Cynthia Rudin, Rich Caruana:
Missing Values and Imputation in Healthcare Data: Can Interpretable Machine Learning Help? CoRR abs/2304.11749 (2023) - [i94]Marco Morucci, Vittorio Orlandi, Harsh Parikh, Sudeepa Roy, Cynthia Rudin, Alexander Volfovsky:
A Double Machine Learning Approach to Combining Experimental and Observational Data. CoRR abs/2307.01449 (2023) - [i93]Pranay Jain, Cheng Ding, Cynthia Rudin, Xiao Hu:
A Self-Supervised Algorithm for Denoising Photoplethysmography Signals for Heart Rate Estimation from Wearables. CoRR abs/2307.05339 (2023) - [i92]Sully F. Chen
, Zhicheng Guo, Cheng Ding, Xiao Hu, Cynthia Rudin:
Learned Kernels for Interpretable and Efficient PPG Signal Quality Assessment and Artifact Segmentation. CoRR abs/2307.05385 (2023) - [i91]Jon Donnelly, Srikar Katta, Cynthia Rudin, Edward P. Browne:
The Rashomon Importance Distribution: Getting RID of Unstable, Single Model-based Variable Importance. CoRR abs/2309.13775 (2023) - [i90]Zhicheng Guo, Cheng Ding, Duc H. Do, Amit J. Shah, Randall J. Lee, Xiao Hu, Cynthia Rudin:
SiamAF: Learning Shared Information from ECG and PPG Signals for Robust Atrial Fibrillation Detection. CoRR abs/2310.09203 (2023) - [i89]Han Zhang, Rayehe Karimi Mahabadi, Cynthia Rudin, Johann Guilleminot, L. Catherine Brinson:
Uncertainty Quantification of Bandgaps in Acoustic Metamaterials with Stochastic Geometric Defects and Material Properties. CoRR abs/2310.12869 (2023) - [i88]Harsh Parikh, Quinn Lanners, Zade Akras, Sahar F. Zafar, M. Brandon Westover, Cynthia Rudin, Alexander Volfovsky:
Estimating Trustworthy and Safe Optimal Treatment Regimes. CoRR abs/2310.15333 (2023) - [i87]Chiyu Ma, Brandon Zhao, Chaofan Chen, Cynthia Rudin:
This Looks Like Those: Illuminating Prototypical Concepts Using Multiple Visualizations. CoRR abs/2310.18589 (2023) - [i86]Lesia Semenova, Harry Chen, Ronald Parr, Cynthia Rudin:
A Path to Simpler Models Starts With Noise. CoRR abs/2310.19726 (2023) - [i85]Chloe Qinyu Zhu, Muhang Tian, Lesia Semenova, Jiachang Liu, Jack Xu, Joseph Scarpa, Cynthia Rudin:
Fast and Interpretable Mortality Risk Scores for Critical Care Patients. CoRR abs/2311.13015 (2023) - [i84]Cheng Ding, Zhicheng Guo, Cynthia Rudin, Ran Xiao, Fadi B. Nahab, Xiao Hu:
Reconsideration on evaluation of machine learning models in continuous monitoring using wearables. CoRR abs/2312.02300 (2023) - [i83]Dennis Tang, Frank Willard, Ronan Tegerdine, Luke Triplett, Jon Donnelly, Luke Moffett, Lesia Semenova, Alina Jade Barnett
, Jin Jing, Cynthia Rudin, M. Brandon Westover:
ProtoEEGNet: An Interpretable Approach for Detecting Interictal Epileptiform Discharges. CoRR abs/2312.10056 (2023) - [i82]Srikar Katta, Harsh Parikh, Cynthia Rudin, Alexander Volfovsky:
Interpretable Causal Inference for Analyzing Wearable, Sensor, and Distributional Data. CoRR abs/2312.10569 (2023) - 2022
- [j47]Chaofan Chen
, Kangcheng Lin, Cynthia Rudin, Yaron Shaposhnik
, Sijia Wang, Tong Wang
:
A holistic approach to interpretability in financial lending: Models, visualizations, and summary-explanations. Decis. Support Syst. 152: 113647 (2022) - [j46]Tong Wang
, Cynthia Rudin
:
Causal Rule Sets for Identifying Subgroups with Enhanced Treatment Effects. INFORMS J. Comput. 34(3): 1626-1643 (2022) - [j45]Chunxiao Li, Cynthia Rudin, Tyler H. McCormick:
Rethinking Nonlinear Instrumental Variable Models through Prediction Validity. J. Mach. Learn. Res. 23: 96:1-96:55 (2022) - [j44]Harsh Parikh, Cynthia Rudin, Alexander Volfovsky:
MALTS: Matching After Learning to Stretch. J. Mach. Learn. Res. 23: 240:1-240:42 (2022) - [c67]Hayden McTavish
, Chudi Zhong, Reto Achermann, Ilias Karimalis
, Jacques Chen, Cynthia Rudin, Margo I. Seltzer:
Fast Sparse Decision Tree Optimization via Reference Ensembles. AAAI 2022: 9604-9613 - [c66]Jiachang Liu, Chudi Zhong, Margo I. Seltzer, Cynthia Rudin:
Fast Sparse Classification for Generalized Linear and Additive Models. AISTATS 2022: 9304-9333 - [c65]Ali Behrouz, Mathias Lécuyer, Cynthia Rudin, Mango I. Seltzer:
Fast optimization of weighted sparse decision trees for use in optimal treatment regimes and optimal policy design. CIKM Workshops 2022 - [c64]Lesia Semenova, Cynthia Rudin, Ronald Parr:
On the Existence of Simpler Machine Learning Models. FAccT 2022: 1827-1858 - [c63]Alina Jade Barnett
, Vaibhav Sharma, Neel Gajjar, Jerry Fang, Fides Regina Schwartz
, Chaofan Chen, Joseph Y. Lo, Cynthia Rudin:
Interpretable deep learning models for better clinician-AI communication in clinical mammography. Image Perception, Observer Performance, and Technology Assessment 2022 - [c62]Jiachang Liu, Chudi Zhong, Boxuan Li, Margo I. Seltzer, Cynthia Rudin:
FasterRisk: Fast and Accurate Interpretable Risk Scores. NeurIPS 2022 - [c61]Rui Xin, Chudi Zhong, Zhi Chen, Takuya Takagi, Margo I. Seltzer, Cynthia Rudin:
Exploring the Whole Rashomon Set of Sparse Decision Trees. NeurIPS 2022 - [c60]Elita A. Lobo, Harvineet Singh, Marek Petrik, Cynthia Rudin, Himabindu Lakkaraju:
Data poisoning attacks on off-policy policy evaluation methods. UAI 2022: 1264-1274 - [c59]Zijie J. Wang, Chudi Zhong, Rui Xin, Takuya Takagi, Zhi Chen, Duen Horng Chau, Cynthia Rudin, Margo I. Seltzer:
TimberTrek: Exploring and Curating Sparse Decision Trees with Interactive Visualization. IEEE VIS (Short Papers) 2022: 60-64 - [i81]Jiachang Liu, Chudi Zhong, Margo I. Seltzer, Cynthia Rudin:
Fast Sparse Classification for Generalized Linear and Additive Models. CoRR abs/2202.11389 (2022) - [i80]Harsh Parikh, Kentaro Hoffman, Haoqi Sun, Wendong Ge, Jin Jing, Rajesh Amerineni, Lin Liu, Jimeng Sun
, Sahar Zafar, Aaron Struck, Alexander Volfovsky, Cynthia Rudin, M. Brandon Westover:
Why Interpretable Causal Inference is Important for High-Stakes Decision Making for Critically Ill Patients and How To Do It. CoRR abs/2203.04920 (2022) - [i79]Haiyang Huang, Zhi Chen, Cynthia Rudin:
SegDiscover: Visual Concept Discovery via Unsupervised Semantic Segmentation. CoRR abs/2204.10926 (2022) - [i78]Yishay Mansour, Michal Moshkovitz, Cynthia Rudin:
There is no Accuracy-Interpretability Tradeoff in Reinforcement Learning for Mazes. CoRR abs/2206.04266 (2022) - [i77]Rui Xin, Chudi Zhong, Zhi Chen, Takuya Takagi, Margo I. Seltzer, Cynthia Rudin:
Exploring the Whole Rashomon Set of Sparse Decision Trees. CoRR abs/2209.08040 (2022) - [i76]Zijie J. Wang, Chudi Zhong, Rui Xin, Takuya Takagi, Zhi Chen, Duen Horng Chau, Cynthia Rudin, Margo I. Seltzer:
TimberTrek: Exploring and Curating Sparse Decision Trees with Interactive Visualization. CoRR abs/2209.09227 (2022) - [i75]Jiachang Liu, Chudi Zhong, Boxuan Li, Margo I. Seltzer, Cynthia Rudin:
FasterRisk: Fast and Accurate Interpretable Risk Scores. CoRR abs/2210.05846 (2022) - [i74]Ali Behrouz, Mathias Lécuyer, Cynthia Rudin, Margo I. Seltzer:
Fast Optimization of Weighted Sparse Decision Trees for use in Optimal Treatment Regimes and Optimal Policy Design. CoRR abs/2210.06825 (2022) - [i73]Alina Jade Barnett
, Zhicheng Guo, Jin Jing, Wendong Ge, Cynthia Rudin, M. Brandon Westover:
Mapping the Ictal-Interictal-Injury Continuum Using Interpretable Machine Learning. CoRR abs/2211.05207 (2022) - [i72]Rui Zhang, Rui Xin, Margo I. Seltzer, Cynthia Rudin:
Optimal Sparse Regression Trees. CoRR abs/2211.14980 (2022) - 2021
- [j43]Divya Koyyalagunta, Anna Y. Sun, Rachel Lea Draelos, Cynthia Rudin:
Playing Codenames with Language Graphs and Word Embeddings. J. Artif. Intell. Res. 71: 319-346 (2021) - [j42]Stefano Tracà, Cynthia Rudin, Weiyu Yan:
Regulating Greed Over Time in Multi-Armed Bandits. J. Mach. Learn. Res. 22: 3:1-3:99 (2021) - [j41]Tianyu Wang
, Marco Morucci, M. Usaid Awan, Yameng Liu, Sudeepa Roy, Cynthia Rudin, Alexander Volfovsky:
FLAME: A Fast Large-scale Almost Matching Exactly Approach to Causal Inference. J. Mach. Learn. Res. 22: 31:1-31:41 (2021) - [j40]Yingfan Wang, Haiyang Huang, Cynthia Rudin, Yaron Shaposhnik:
Understanding How Dimension Reduction Tools Work: An Empirical Approach to Deciphering t-SNE, UMAP, TriMap, and PaCMAP for Data Visualization. J. Mach. Learn. Res. 22: 201:1-201:73 (2021) - [j39]Beau Coker
, Cynthia Rudin
, Gary King
:
A Theory of Statistical Inference for Ensuring the Robustness of Scientific Results. Manag. Sci. 67(10): 6174-6197 (2021) - [j38]Alina Jade Barnett
, Fides Regina Schwartz
, Chaofan Tao, Chaofan Chen
, Yinhao Ren, Joseph Y. Lo, Cynthia Rudin
:
A case-based interpretable deep learning model for classification of mass lesions in digital mammography. Nat. Mach. Intell. 3(12): 1061-1070 (2021) - [j37]Jianyou Wang, Cynthia Rudin, Yuren Zhou, Christopher Suh, Xiaoxuan Zhang:
There Once Was a Really Bad Poet, It Was Automated but You Didn't Know It. Trans. Assoc. Comput. Linguistics 9: 605-620 (2021) - [c58]Michael Anis Mihdi Afnan, Cynthia Rudin, Vincent Conitzer, Julian Savulescu, Abhishek Mishra
, Yanhe Liu, Masoud Afnan:
Ethical Implementation of Artificial Intelligence to Select Embryos in In Vitro Fertilization. AIES 2021: 316-326 - [d1]Alexander C. Ogren, Zhi Chen, L. Catherine Brinson, Mary Bastawrous, Cynthia Rudin, Chiara Daraio:
2D elastodynamic metamaterials. UCI Machine Learning Repository, 2021 - [i71]Neha R. Gupta, Vittorio Orlandi, Chia-Rui Chang, Tianyu Wang, Marco Morucci, Pritam Dey, Thomas J. Howell, Xian Sun, Angikar Ghosal, Sudeepa Roy, Cynthia Rudin, Alexander Volfovsky:
dame-flame: A Python Library Providing Fast Interpretable Matching for Causal Inference. CoRR abs/2101.01867 (2021) - [i70]Jianyou Wang, Xiaoxuan Zhang, Yuren Zhou, Christopher Suh, Cynthia Rudin:
There Once Was a Really Bad Poet, It Was Automated but You Didn't Know It. CoRR abs/2103.03775 (2021) - [i69]Cynthia Rudin, Chaofan Chen, Zhi Chen, Haiyang Huang, Lesia Semenova, Chudi Zhong:
Interpretable Machine Learning: Fundamental Principles and 10 Grand Challenges. CoRR abs/2103.11251 (2021) - [i68]Alina Jade Barnett, Fides Regina Schwartz, Chaofan Tao, Chaofan Chen, Yinhao Ren, Joseph Y. Lo, Cynthia Rudin:
IAIA-BL: A Case-based Interpretable Deep Learning Model for Classification of Mass Lesions in Digital Mammography. CoRR abs/2103.12308 (2021) - [i67]Michael Anis Mihdi Afnan, Cynthia Rudin, Vincent Conitzer, Julian Savulescu, Abhishek Mishra, Yanhe Liu, Masoud Afnan:
Ethical Implementation of Artificial Intelligence to Select Embryos in In Vitro Fertilization. CoRR abs/2105.00060 (2021) - [i66]Divya Koyyalagunta, Anna Y. Sun, Rachel Lea Draelos, Cynthia Rudin:
Playing Codenames with Language Graphs and Word Embeddings. CoRR abs/2105.05885 (2021) - [i65]Chaofan Chen, Kangcheng Lin, Cynthia Rudin, Yaron Shaposhnik, Sijia Wang, Tong Wang:
A Holistic Approach to Interpretability in Financial Lending: Models, Visualizations, and Summary-Explanations. CoRR abs/2106.02605 (2021) - [i64]Alex Oesterling, Angikar Ghosal, Haoyang Yu, Rui Xin, Yasa Baig, Lesia Semenova, Cynthia Rudin:
Multitask Learning for Citation Purpose Classification. CoRR abs/2106.13275 (2021) - [i63]Alina Jade Barnett, Fides Regina Schwartz, Chaofan Tao, Chaofan Chen, Yinhao Ren, Joseph Y. Lo, Cynthia Rudin:
Interpretable Mammographic Image Classification using Cased-Based Reasoning and Deep Learning. CoRR abs/2107.05605 (2021) - [i62]Yunyao Zhu, Stephen Hahn, Simon Mak, Yue Jiang, Cynthia Rudin:
BacHMMachine: An Interpretable and Scalable Model for Algorithmic Harmonization for Four-part Baroque Chorales. CoRR abs/2109.07623 (2021) - [i61]Zhi Chen, Alexander C. Ogren, Chiara Daraio, L. Catherine Brinson, Cynthia Rudin:
How to See Hidden Patterns in Metamaterials with Interpretable Machine Learning. CoRR abs/2111.05949 (2021) - [i60]Hayden McTavish, Chudi Zhong, Reto Achermann, Ilias Karimalis, Jacques Chen, Cynthia Rudin, Margo I. Seltzer:
Fast Sparse Decision Tree Optimization via Reference Ensembles. CoRR abs/2112.00798 (2021) - 2020
- [j36]Alexander S. Rich, Cynthia Rudin, David M. P. Jacoby, Robin Freeman, Oliver R. Wearn, Henry Shevlin, Kanta Dihal, Seán S. ÓhÉigeartaigh, James Butcher, Marco Lippi, Przemyslaw Palka, Paolo Torroni
, Shannon Wongvibulsin, Edmon Begoli, Gisbert Schneider, Stephen Cave, Mona Sloane, Emanuel Moss, Iyad Rahwan, Ken Goldberg, David Howard, Luciano Floridi, Jack Stilgoe:
AI reflections in 2019. Nat. Mach. Intell. 2(1): 2-9 (2020) - [j35]Zhi Chen
, Yijie Bei
, Cynthia Rudin
:
Concept whitening for interpretable image recognition. Nat. Mach. Intell. 2(12): 772-782 (2020) - [j34]Jiayun Dong
, Cynthia Rudin
:
Exploring the cloud of variable importance for the set of all good models. Nat. Mach. Intell. 2(12): 810-824 (2020) - [c57]Jerry Liu, Nathan O'Hara, Alexander Rubin, Rachel Lea Draelos
, Cynthia Rudin:
Metaphor Detection Using Contextual Word Embeddings From Transformers. Fig-Lang@ACL 2020: 250-255 - [c56]Hunter Gregory, Steven Li, Pouya Mohammadi, Natalie Tarn, Rachel Lea Draelos
, Cynthia Rudin:
A Transformer Approach to Contextual Sarcasm Detection in Twitter. Fig-Lang@ACL 2020: 270-275 - [c55]M. Usaid Awan, Marco Morucci, Vittorio Orlandi, Sudeepa Roy, Cynthia Rudin, Alexander Volfovsky:
Almost-Matching-Exactly for Treatment Effect Estimation under Network Interference. AISTATS 2020: 3252-3262 - [c54]Sachit Menon, Alexandru Damian, Shijia Hu, Nikhil Ravi, Cynthia Rudin:
PULSE: Self-Supervised Photo Upsampling via Latent Space Exploration of Generative Models. CVPR 2020: 2434-2442 - [c53]Tianyu Wang
, Weicheng Ye, Dawei Geng, Cynthia Rudin:
Towards Practical Lipschitz Bandits. FODS 2020: 129-138 - [c52]Jimmy Lin, Chudi Zhong, Diane Hu, Cynthia Rudin, Margo I. Seltzer:
Generalized and Scalable Optimal Sparse Decision Trees. ICML 2020: 6150-6160 - [c51]Tianyu Wang, Cynthia Rudin:
Bandits for BMO Functions. ICML 2020: 9996-10006 - [c50]Marco Morucci, Vittorio Orlandi, Sudeepa Roy, Cynthia Rudin, Alexander Volfovsky:
Adaptive Hyper-box Matching for Interpretable Individualized Treatment Effect Estimation. UAI 2020: 1089-1098 - [i59]Zhi Chen, Yijie Bei, Cynthia Rudin:
Concept Whitening for Interpretable Image Recognition. CoRR abs/2002.01650 (2020) - [i58]M. Usaid Awan, Marco Morucci, Vittorio Orlandi, Sudeepa Roy, Cynthia Rudin, Alexander Volfovsky:
Almost-Matching-Exactly for Treatment Effect Estimation under Network Interference. CoRR abs/2003.00964 (2020) - [i57]Marco Morucci, Vittorio Orlandi, Sudeepa Roy, Cynthia Rudin, Alexander Volfovsky:
Adaptive Hyper-box Matching for Interpretable Individualized Treatment Effect Estimation. CoRR abs/2003.01805 (2020) - [i56]Sachit Menon, Alexandru Damian, Shijia Hu, Nikhil Ravi, Cynthia Rudin:
PULSE: Self-Supervised Photo Upsampling via Latent Space Exploration of Generative Models. CoRR abs/2003.03808 (2020) - [i55]Caroline Wang, Bin Han, Bhrij Patel, Feroze Mohideen, Cynthia Rudin:
In Pursuit of Interpretable, Fair and Accurate Machine Learning for Criminal Recidivism Prediction. CoRR abs/2005.04176 (2020) - [i54]Jimmy Lin, Chudi Zhong, Diane Hu, Cynthia Rudin, Margo I. Seltzer:
Generalized Optimal Sparse Decision Trees. CoRR abs/2006.08690 (2020) - [i53]Tianyu Wang, Cynthia Rudin:
Bandits for BMO Functions. CoRR abs/2007.08703 (2020) - [i52]Qinwen Huang, Ye Zhou, Xiaochen Du, Reed Chen, Jianyou Wang, Cynthia Rudin, Alberto Bartesaghi:
Cryo-ZSSR: multiple-image super-resolution based on deep internal learning. CoRR abs/2011.11020 (2020) - [i51]Yingfan Wang, Haiyang Huang, Cynthia Rudin, Yaron Shaposhnik:
Understanding How Dimension Reduction Tools Work: An Empirical Approach to Deciphering t-SNE, UMAP, TriMAP, and PaCMAP for Data Visualization. CoRR abs/2012.04456 (2020)
2010 – 2019
- 2019
- [j33]Gah-Yi Ban
, Cynthia Rudin
:
The Big Data Newsvendor: Practical Insights from Machine Learning. Oper. Res. 67(1): 90-108 (2019) - [j32]Berk Ustun
, Cynthia Rudin:
Learning Optimized Risk Scores. J. Mach. Learn. Res. 20: 150:1-150:75 (2019) - [j31]Aaron Fisher, Cynthia Rudin, Francesca Dominici:
All Models are Wrong, but Many are Useful: Learning a Variable's Importance by Studying an Entire Class of Prediction Models Simultaneously. J. Mach. Learn. Res. 20: 177:1-177:81 (2019) - [j30]Cynthia Rudin
:
Stop explaining black box machine learning models for high stakes decisions and use interpretable models instead. Nat. Mach. Intell. 1(5): 206-215 (2019) - [c49]Awa Dieng, Yameng Liu, Sudeepa Roy, Cynthia Rudin, Alexander Volfovsky:
Interpretable Almost-Exact Matching for Causal Inference. AISTATS 2019: 2445-2453 - [c48]Peter Hase, Chaofan Chen, Oscar Li, Cynthia Rudin:
Interpretable Image Recognition with Hierarchical Prototypes. HCOMP 2019: 32-40 - [c47]Cynthia Rudin:
Do Simpler Models Exist and How Can We Find Them? KDD 2019: 1-2 - [c46]Xiyang Hu, Cynthia Rudin, Margo I. Seltzer:
Optimal Sparse Decision Trees. NeurIPS 2019: 7265-7273 - [c45]Chaofan Chen, Oscar Li, Daniel Tao, Alina Barnett, Cynthia Rudin, Jonathan Su:
This Looks Like That: Deep Learning for Interpretable Image Recognition. NeurIPS 2019: 8928-8939 - [c44]Stefano Tracà, Weiyu Yan, Cynthia Rudin:
Reducing Exploration of Dying Arms in Mortal Bandits. UAI 2019: 156-163 - [c43]M. Usaid Awan, Yameng Liu, Marco Morucci, Sudeepa Roy, Cynthia Rudin, Alexander Volfovsky:
Interpretable Almost Matching Exactly With Instrumental Variables. UAI 2019: 1116-1126 - [i50]Jiayun Dong, Cynthia Rudin:
Variable Importance Clouds: A Way to Explore Variable Importance for the Set of Good Models. CoRR abs/1901.03209 (2019) - [i49]Tianyu Wang, Dawei Geng, Cynthia Rudin:
A Practical Bandit Method with Advantages in Neural Network Tuning. CoRR abs/1901.09277 (2019) - [i48]Xiyang Hu
, Cynthia Rudin, Margo I. Seltzer:
Optimal Sparse Decision Trees. CoRR abs/1904.12847 (2019) - [i47]Cynthia Rudin, David Carlson:
The Secrets of Machine Learning: Ten Things You Wish You Had Known Earlier to be More Effective at Data Analysis. CoRR abs/1906.01998 (2019) - [i46]Peter Hase, Chaofan Chen, Oscar Li, Cynthia Rudin:
Interpretable Image Recognition with Hierarchical Prototypes. CoRR abs/1906.10651 (2019) - [i45]M. Usaid Awan, Yameng Liu, Marco Morucci, Sudeepa Roy, Cynthia Rudin, Alexander Volfovsky:
Interpretable Almost-Matching-Exactly With Instrumental Variables. CoRR abs/1906.11658 (2019) - [i44]Stefano Tracà, Cynthia Rudin, Weiyu Yan:
Reducing Exploration of Dying Arms in Mortal Bandits. CoRR abs/1907.02571 (2019) - [i43]Lesia Semenova, Cynthia Rudin:
A study in Rashomon curves and volumes: A new perspective on generalization and model simplicity in machine learning. CoRR abs/1908.01755 (2019) - 2018
- [j29]Cynthia Rudin
, Berk Ustun:
Optimized Scoring Systems: Toward Trust in Machine Learning for Healthcare and Criminal Justice. Interfaces 48(5): 449-466 (2018) - [j28]Cynthia Rudin, Seyda Ertekin:
Learning customized and optimized lists of rules with mathematical programming. Math. Program. Comput. 10(4): 659-702 (2018) - [c42]Oscar Li, Hao Liu, Chaofan Chen, Cynthia Rudin:
Deep Learning for Case-Based Reasoning Through Prototypes: A Neural Network That Explains Its Predictions. AAAI 2018: 3530-3537 - [c41]Chaofan Chen, Cynthia Rudin:
An Optimization Approach to Learning Falling Rule Lists. AISTATS 2018: 604-612 - [c40]Cynthia Rudin, Yining Wang:
Direct Learning to Rank And Rerank. AISTATS 2018: 775-783 - [c39]Yijie Bei, Alexandru Damian, Shijia Hu, Sachit Menon, Nikhil Ravi, Cynthia Rudin:
New Techniques for Preserving Global Structure and Denoising With Low Information Loss in Single-Image Super-Resolution. CVPR Workshops 2018: 874-881 - [i42]Cynthia Rudin, Yining Wang:
Direct Learning to Rank and Rerank. CoRR abs/1802.07400 (2018) - [i41]Siong Thye Goh, Cynthia Rudin:
A Minimax Surrogate Loss Approach to Conditional Difference Estimation. CoRR abs/1803.03769 (2018) - [i40]Beau Coker, Cynthia Rudin, Gary King:
A Theory of Statistical Inference for Ensuring the Robustness of Scientific Results. CoRR abs/1804.08646 (2018) - [i39]Yijie Bei, Alexandru Damian, Shijia Hu, Sachit Menon, Nikhil Ravi, Cynthia Rudin:
New Techniques for Preserving Global Structure and Denoising with Low Information Loss in Single-Image Super-Resolution. CoRR abs/1805.03383 (2018) - [i38]Awa Dieng, Yameng Liu, Sudeepa Roy, Cynthia Rudin, Alexander Volfovsky:
Collapsing-Fast-Large-Almost-Matching-Exactly: A Matching Method for Causal Inference. CoRR abs/1806.06802 (2018) - [i37]Chaofan Chen, Oscar Li, Alina Barnett, Jonathan Su, Cynthia Rudin:
This looks like that: deep learning for interpretable image recognition. CoRR abs/1806.10574 (2018) - [i36]Ramin Moghaddass, Cynthia Rudin:
Bayesian Patchworks: An Approach to Case-Based Reasoning. CoRR abs/1809.03541 (2018) - [i35]Cynthia Rudin, Caroline Wang, Beau Coker:
The age of secrecy and unfairness in recidivism prediction. CoRR abs/1811.00731 (2018) - [i34]John Benhart, Tianlin Duan, Peter Hase, Liuyi Zhu, Cynthia Rudin:
Shall I Compare Thee to a Machine-Written Sonnet? An Approach to Algorithmic Sonnet Generation. CoRR abs/1811.05067 (2018) - [i33]Harsh Parikh, Cynthia Rudin, Alexander Volfovsky:
MALTS: Matching After Learning to Stretch. CoRR abs/1811.07415 (2018) - [i32]Cynthia Rudin:
Please Stop Explaining Black Box Models for High Stakes Decisions. CoRR abs/1811.10154 (2018) - [i31]Chaofan Chen, Kangcheng Lin, Cynthia Rudin, Yaron Shaposhnik, Sijia Wang, Tong Wang:
An Interpretable Model with Globally Consistent Explanations for Credit Risk. CoRR abs/1811.12615 (2018) - 2017
- [j27]Tong Wang, Cynthia Rudin, Finale Doshi-Velez, Yimin Liu, Erica Klampfl, Perry MacNeille:
A Bayesian Framework for Learning Rule Sets for Interpretable Classification. J. Mach. Learn. Res. 18: 70:1-70:37 (2017) - [j26]Elaine Angelino, Nicholas Larus-Stone, Daniel Alabi, Margo I. Seltzer, Cynthia Rudin:
Learning Certifiably Optimal Rule Lists for Categorical Data. J. Mach. Learn. Res. 18: 234:1-234:78 (2017) - [c38]Himabindu Lakkaraju, Cynthia Rudin:
Learning Cost-Effective and Interpretable Treatment Regimes. AISTATS 2017: 166-175 - [c37]Hongyu Yang, Cynthia Rudin, Margo I. Seltzer:
Scalable Bayesian Rule Lists. ICML 2017: 3921-3930 - [c36]Elaine Angelino, Nicholas Larus-Stone, Daniel Alabi, Margo I. Seltzer, Cynthia Rudin:
Learning Certifiably Optimal Rule Lists. KDD 2017: 35-44 - [c35]Berk Ustun
, Cynthia Rudin:
Optimized Risk Scores. KDD 2017: 1125-1134 - [i30]Elaine Angelino, Nicholas Larus-Stone, Daniel Alabi, Margo I. Seltzer, Cynthia Rudin:
Learning Certifiably Optimal Rule Lists for Categorical Data. CoRR abs/1704.01701 (2017) - [i29]Sudeepa Roy, Cynthia Rudin, Alexander Volfovsky, Tianyu Wang:
FLAME: A Fast Large-scale Almost Matching Exactly Approach to Causal Inference. CoRR abs/1707.06315 (2017) - [i28]Chaofan Chen, Cynthia Rudin:
An optimization approach to learning falling rule lists. CoRR abs/1710.02572 (2017) - [i27]Oscar Li, Hao Liu, Chaofan Chen, Cynthia Rudin:
Deep Learning for Case-based Reasoning through Prototypes: A Neural Network that Explains its Predictions. CoRR abs/1710.04806 (2017) - [i26]Tong Wang, Cynthia Rudin:
Causal Rule Sets for Identifying Subgroups with Enhanced Treatment Effect. CoRR abs/1710.05426 (2017) - [i25]Fulton Wang, Cynthia Rudin:
Dimension Reduction for Robust Covariate Shift Correction. CoRR abs/1711.10938 (2017) - 2016
- [j25]Ramin Moghaddass, Cynthia Rudin, David Madigan:
The Factorized Self-Controlled Case Series Method: An Approach for Estimating the Effects of Many Drugs on Many Outcomes. J. Mach. Learn. Res. 17: 185:1-185:24 (2016) - [j24]Berk Ustun
, Cynthia Rudin:
Supersparse linear integer models for optimized medical scoring systems. Mach. Learn. 102(3): 349-391 (2016) - [j23]William Souillard-Mandar, Randall Davis, Cynthia Rudin, Rhoda Au, David J. Libon, Rodney Swenson, Catherine C. Price
, Melissa Lamar, Dana L. Penney:
Learning classification models of cognitive conditions from subtle behaviors in the digital Clock Drawing Test. Mach. Learn. 102(3): 393-441 (2016) - [c34]Vikas K. Garg, Cynthia Rudin, Tommi S. Jaakkola:
CRAFT: ClusteR-specific Assorted Feature selecTion. AISTATS 2016: 305-313 - [c33]Tong Wang, Cynthia Rudin, Finale Doshi-Velez, Yimin Liu, Erica Klampfl, Perry MacNeille:
Bayesian Rule Sets for Interpretable Classification. ICDM 2016: 1269-1274 - [c32]Benjamin Letham, Lydia M. Letham, Cynthia Rudin:
Bayesian Inference of Arrival Rate and Substitution Behavior from Sales Transaction Data with Stockouts. KDD 2016: 1695-1704 - [i24]Hongyu Yang, Cynthia Rudin, Margo I. Seltzer:
Scalable Bayesian Rule Lists. CoRR abs/1602.08610 (2016) - [i23]William Souillard-Mandar, Randall Davis, Cynthia Rudin, Rhoda Au, Dana L. Penney:
Interpretable Machine Learning Models for the Digital Clock Drawing Test. CoRR abs/1606.07163 (2016) - [i22]Himabindu Lakkaraju, Cynthia Rudin:
Learning Cost-Effective Treatment Regimes using Markov Decision Processes. CoRR abs/1610.06972 (2016) - 2015
- [j22]Tong Wang, Cynthia Rudin, Daniel Wagner, Rich Sevieri:
Finding Patterns with a Rotten Core: Data Mining for Crime Series with Cores. Big Data 3(1): 3-21 (2015) - [j21]Seyda Ertekin, Cynthia Rudin:
A Bayesian Approach to Learning Scoring Systems. Big Data 3(4): 267-276 (2015) - [j20]Theja Tulabandhula, Cynthia Rudin:
Generalization bounds for learning with linear, polygonal, quadratic and conic side knowledge. Mach. Learn. 100(2-3): 183-216 (2015) - [c31]Fulton Wang, Cynthia Rudin:
Falling Rule Lists. AISTATS 2015 - [i21]Berk Ustun, Cynthia Rudin:
Supersparse Linear Integer Models for Optimized Medical Scoring Systems. CoRR abs/1502.04269 (2015) - [i20]Been Kim, Cynthia Rudin, Julie A. Shah:
The Bayesian Case Model: A Generative Approach for Case-Based Reasoning and Prototype Classification. CoRR abs/1503.01161 (2015) - [i19]Tong Wang, Cynthia Rudin, Finale Doshi-Velez, Yimin Liu, Erica Klampfl, Perry MacNeille:
Or's of And's for Interpretable Classification, with Application to Context-Aware Recommender Systems. CoRR abs/1504.07614 (2015) - [i18]Stefano Tracà, Cynthia Rudin:
Regulating Greed Over Time. CoRR abs/1505.05629 (2015) - [i17]Vikas K. Garg, Cynthia Rudin, Tommi S. Jaakkola:
CRAFT: ClusteR-specific Assorted Feature selecTion. CoRR abs/1506.07609 (2015) - [i16]Fulton Wang, Cynthia Rudin:
Causal Falling Rule Lists. CoRR abs/1510.05189 (2015) - [i15]Benjamin Letham, Cynthia Rudin, Tyler H. McCormick, David Madigan:
Interpretable classifiers using rules and Bayesian analysis: Building a better stroke prediction model. CoRR abs/1511.01644 (2015) - [i14]Tong Wang, Cynthia Rudin:
Learning Optimized Or's of And's. CoRR abs/1511.02210 (2015) - 2014
- [j19]Theja Tulabandhula, Cynthia Rudin:
Tire Changes, Fresh Air, and Yellow Flags: Challenges in Predictive Analytics for Professional Racing. Big Data 2(2): 97-112 (2014) - [j18]Been Kim, Cynthia Rudin:
Learning about meetings. Data Min. Knowl. Discov. 28(5-6): 1134-1157 (2014) - [j17]Seyda Ertekin, Cynthia Rudin, Haym Hirsh:
Approximating the crowd. Data Min. Knowl. Discov. 28(5-6): 1189-1221 (2014) - [j16]Cynthia Rudin, Seyda Ertekin, Rebecca J. Passonneau, Axinia Radeva, Ashish Tomar, Boyi Xie, Stanley Lewis, Mark Riddle, Debbie Pangsrivinij, Tyler H. McCormick
:
Analytics for Power Grid Distribution Reliability in New York City. Interfaces 44(4): 364-383 (2014) - [j15]Cynthia Rudin, Kiri L. Wagstaff
:
Machine learning for science and society. Mach. Learn. 95(1): 1-9 (2014) - [j14]Theja Tulabandhula, Cynthia Rudin:
On combining machine learning with decision making. Mach. Learn. 97(1-2): 33-64 (2014) - [c30]Dingquan Wang, Rebecca J. Passonneau, Michael Collins, Cynthia Rudin:
Modeling Weather Impact on a Secondary Electrical Grid. ANT/SEIT 2014: 631-638 - [c29]Jonathan H. Huggins, Cynthia Rudin:
Toward a Theory of Pattern Discovery. ISAIM 2014 - [c28]Theja Tulabandhula, Cynthia Rudin:
Generalization Bounds for Learning with Linear and Quadratic Side Knowledge. ISAIM 2014 - [c27]Theja Tulabandhula, Cynthia Rudin:
Robust Optimization using Machine Learning for Uncertainty Sets. ISAIM 2014 - [c26]Siong Thye Goh
, Cynthia Rudin:
Box drawings for learning with imbalanced data. KDD 2014: 333-342 - [c25]Cynthia Rudin:
Algorithms for interpretable machine learning. KDD 2014: 1519 - [c24]Been Kim, Cynthia Rudin, Julie A. Shah:
The Bayesian Case Model: A Generative Approach for Case-Based Reasoning and Prototype Classification. NIPS 2014: 1952-1960 - [c23]Jonathan H. Huggins, Cynthia Rudin:
A Statistical Learning Theory Framework for Supervised Pattern Discovery. SDM 2014: 506-514 - [i13]Siong Thye Goh, Cynthia Rudin:
Box Drawings for Learning with Imbalanced Data. CoRR abs/1403.3378 (2014) - [i12]Berk Ustun, Cynthia Rudin:
Methods and Models for Interpretable Linear Classification. CoRR abs/1405.4047 (2014) - [i11]Theja Tulabandhula, Cynthia Rudin:
Generalization Bounds for Learning with Linear, Polygonal, Quadratic and Conic Side Knowledge. CoRR abs/1405.7764 (2014) - [i10]Theja Tulabandhula, Cynthia Rudin:
Robust Optimization using Machine Learning for Uncertainty Sets. CoRR abs/1407.1097 (2014) - [i9]Fulton Wang, Cynthia Rudin:
Falling Rule Lists. CoRR abs/1411.5899 (2014) - [i8]Johannes Fürnkranz, Eyke Hüllermeier, Cynthia Rudin, Roman Slowinski, Scott Sanner:
Preference Learning (Dagstuhl Seminar 14101). Dagstuhl Reports 4(3): 1-27 (2014) - 2013
- [j13]Benjamin Letham, Cynthia Rudin, Katherine A. Heller:
Growing a list. Data Min. Knowl. Discov. 27(3): 372-395 (2013) - [j12]Theja Tulabandhula, Cynthia Rudin:
Machine learning with operational costs. J. Mach. Learn. Res. 14(1): 1989-2028 (2013) - [j11]Indraneel Mukherjee, Cynthia Rudin, Robert E. Schapire:
The rate of convergence of AdaBoost. J. Mach. Learn. Res. 14(1): 2315-2347 (2013) - [j10]Cynthia Rudin, Benjamin Letham, David Madigan:
Learning theory analysis for association rules and sequential event prediction. J. Mach. Learn. Res. 14(1): 3441-3492 (2013) - [j9]Benjamin Letham, Cynthia Rudin, David Madigan:
Sequential event prediction. Mach. Learn. 93(2-3): 357-380 (2013) - [c22]Seyda Ertekin, Cynthia Rudin, Tyler H. McCormick:
Predicting Power Failures with Reactive Point Processes. AAAI (Late-Breaking Developments) 2013 - [c21]Been Kim, Cynthia Rudin:
Machine Learning for Meeting Analysis. AAAI (Late-Breaking Developments) 2013 - [c20]Benjamin Letham, Cynthia Rudin, Tyler H. McCormick, David Madigan:
An Interpretable Stroke Prediction Model using Rules and Bayesian Analysis. AAAI (Late-Breaking Developments) 2013 - [c19]Berk Ustun, Stefano Tracà, Cynthia Rudin:
Supersparse Linear Integer Models for Predictive Scoring Systems. AAAI (Late-Breaking Developments) 2013 - [c18]Tong Wang, Cynthia Rudin, Daniel Wagner, Rich Sevieri:
Detecting Patterns of Crime with Series Finder. AAAI (Late-Breaking Developments) 2013 - [c17]Tong Wang, Cynthia Rudin, Daniel Wagner, Rich Sevieri:
Learning to Detect Patterns of Crime. ECML/PKDD (3) 2013: 515-530 - [i7]Been Kim, Cynthia Rudin:
Learning About Meetings. CoRR abs/1306.1927 (2013) - [i6]Jonathan H. Huggins, Cynthia Rudin:
Toward a Theory of Pattern Discovery. CoRR abs/1307.0802 (2013) - 2012
- [j8]Allison Chang, Cynthia Rudin, Michael Cavaretta, Robert Thomas, Gloria Chou:
How to reverse-engineer quality rankings. Mach. Learn. 88(3): 369-398 (2012) - [j7]Cynthia Rudin, David L. Waltz, Roger Anderson, Albert Boulanger
, Ansaf Salleb-Aouissi, Maggie Chow, Haimonti Dutta, Philip Gross, Bert Huang, Steve Ierome:
Machine Learning for theNew York City Power Grid. IEEE Trans. Pattern Anal. Mach. Intell. 34(2): 328-345 (2012) - [c16]Seyda Ertekin, Haym Hirsh, Cynthia Rudin:
Selective Sampling of Labelers for Approximating the Crowd. AAAI Fall Symposium: Machine Aggregation of Human Judgment 2012 - [c15]Cynthia Rudin, Theja Tulabandhula:
The Influence of Operational Cost on Estimation. ISAIM 2012 - [c14]Allison Chang, Dimitris Bertsimas, Cynthia Rudin:
An Integer Optimization Approach to Associative Classification. NIPS 2012: 269-277 - [c13]Boyi Xie, Rebecca J. Passonneau, Haimonti Dutta, Jing-Yeu Miaw, Axinia Radeva, Ashish Tomar, Cynthia Rudin:
Progressive Clustering with Learned Seeds: An Event Categorization System for Power Grid. SEKE 2012: 100-105 - [c12]Cynthia Rudin, Robert E. Schapire, Ingrid Daubechies:
Open Problem: Does AdaBoost Always Cycle? COLT 2012: 46.1-46.4 - [i5]Seyda Ertekin, Haym Hirsh, Cynthia Rudin:
Learning to Predict the Wisdom of Crowds. CoRR abs/1204.3611 (2012) - 2011
- [j6]Cynthia Rudin, Rebecca J. Passonneau, Axinia Radeva, Steve Ierome, Delfina Isaac:
21st-Century Data Miners Meet 19th-Century Electrical Cables. Computer 44(6): 103-105 (2011) - [j5]Seyda Ertekin, Cynthia Rudin:
On Equivalence Relationships Between Classification and Ranking Algorithms. J. Mach. Learn. Res. 12: 2905-2929 (2011) - [c11]Theja Tulabandhula, Cynthia Rudin, Patrick Jaillet:
The Machine Learning and Traveling Repairman Problem. ADT 2011: 262-276 - [c10]Indraneel Mukherjee, Cynthia Rudin, Robert E. Schapire:
The Rate of Convergence of Adaboost. COLT 2011: 537-558 - [c9]Cynthia Rudin, Benjamin Letham, Ansaf Salleb-Aouissi, Eugene Kogan, David Madigan:
Sequential Event Prediction with Association Rules. COLT 2011: 615-634 - [i4]Theja Tulabandhula, Cynthia Rudin, Patrick Jaillet:
Machine Learning and the Traveling Repairman. CoRR abs/1104.5061 (2011) - [i3]Indraneel Mukherjee, Cynthia Rudin, Robert E. Schapire:
The Rate of Convergence of AdaBoost. CoRR abs/1106.6024 (2011) - [i2]Theja Tulabandhula, Cynthia Rudin:
Machine Learning with Operational Costs. CoRR abs/1112.0698 (2011) - 2010
- [j4]Cynthia Rudin, Rebecca J. Passonneau, Axinia Radeva, Haimonti Dutta, Steve Ierome, Delfina Isaac:
A process for predicting manhole events in Manhattan. Mach. Learn. 80(1): 1-31 (2010)
2000 – 2009
- 2009
- [j3]Cynthia Rudin, Robert E. Schapire:
Margin-based Ranking and an Equivalence between AdaBoost and RankBoost. J. Mach. Learn. Res. 10: 2193-2232 (2009) - [j2]Cynthia Rudin:
The P-Norm Push: A Simple Convex Ranking Algorithm that Concentrates at the Top of the List. J. Mach. Learn. Res. 10: 2233-2271 (2009) - [c8]Rebecca J. Passonneau, Cynthia Rudin, Axinia Radeva, Zhi An Liu:
Reducing Noise in Labels and Features for a Real World Dataset: Application of NLP Corpus Annotation Methods. CICLing 2009: 86-97 - [c7]Raphael Pelossof, Michael Jones, Ilia Vovsha, Cynthia Rudin:
Online coordinate boosting. ICCV Workshops 2009: 1354-1361 - [c6]Axinia Radeva, Cynthia Rudin, Rebecca J. Passonneau, Delfina Isaac:
Report Cards for Manholes: Eliciting Expert Feedback for a Learning Task. ICMLA 2009: 719-724 - 2008
- [c5]Ryan Roth, Owen Rambow, Nizar Habash, Mona T. Diab, Cynthia Rudin:
Arabic Morphological Tagging, Diacritization, and Lemmatization Using Lexeme Models and Feature Ranking. ACL (2) 2008: 117-120 - 2006
- [c4]Cynthia Rudin:
Ranking with a P-Norm Push. COLT 2006: 589-604 - 2005
- [c3]Cynthia Rudin, Corinna Cortes, Mehryar Mohri, Robert E. Schapire:
Margin-Based Ranking Meets Boosting in the Middle. COLT 2005: 63-78 - [i1]Cynthia Rudin:
Stability Analysis for Regularized Least Squares Regression. CoRR abs/cs/0502016 (2005) - 2004
- [j1]Cynthia Rudin, Ingrid Daubechies, Robert E. Schapire:
The Dynamics of AdaBoost: Cyclic Behavior and Convergence of Margins. J. Mach. Learn. Res. 5: 1557-1595 (2004) - [c2]Cynthia Rudin, Robert E. Schapire, Ingrid Daubechies:
Boosting Based on a Smooth Margin. COLT 2004: 502-517 - 2003
- [c1]Cynthia Rudin, Ingrid Daubechies, Robert E. Schapire:
On the Dynamics of Boosting. NIPS 2003: 1101-1108
Coauthor Index

manage site settings
To protect your privacy, all features that rely on external API calls from your browser are turned off by default. You need to opt-in for them to become active. All settings here will be stored as cookies with your web browser. For more information see our F.A.Q.
Unpaywalled article links
Add open access links from to the list of external document links (if available).
Privacy notice: By enabling the option above, your browser will contact the API of unpaywall.org to load hyperlinks to open access articles. Although we do not have any reason to believe that your call will be tracked, we do not have any control over how the remote server uses your data. So please proceed with care and consider checking the Unpaywall privacy policy.
Archived links via Wayback Machine
For web page which are no longer available, try to retrieve content from the of the Internet Archive (if available).
Privacy notice: By enabling the option above, your browser will contact the API of archive.org to check for archived content of web pages that are no longer available. Although we do not have any reason to believe that your call will be tracked, we do not have any control over how the remote server uses your data. So please proceed with care and consider checking the Internet Archive privacy policy.
Reference lists
Add a list of references from ,
, and
to record detail pages.
load references from crossref.org and opencitations.net
Privacy notice: By enabling the option above, your browser will contact the APIs of crossref.org, opencitations.net, and semanticscholar.org to load article reference information. Although we do not have any reason to believe that your call will be tracked, we do not have any control over how the remote server uses your data. So please proceed with care and consider checking the Crossref privacy policy and the OpenCitations privacy policy, as well as the AI2 Privacy Policy covering Semantic Scholar.
Citation data
Add a list of citing articles from and
to record detail pages.
load citations from opencitations.net
Privacy notice: By enabling the option above, your browser will contact the API of opencitations.net and semanticscholar.org to load citation information. Although we do not have any reason to believe that your call will be tracked, we do not have any control over how the remote server uses your data. So please proceed with care and consider checking the OpenCitations privacy policy as well as the AI2 Privacy Policy covering Semantic Scholar.
OpenAlex data
Load additional information about publications from .
Privacy notice: By enabling the option above, your browser will contact the API of openalex.org to load additional information. Although we do not have any reason to believe that your call will be tracked, we do not have any control over how the remote server uses your data. So please proceed with care and consider checking the information given by OpenAlex.
last updated on 2025-04-20 23:45 CEST by the dblp team
all metadata released as open data under CC0 1.0 license
see also: Terms of Use | Privacy Policy | Imprint